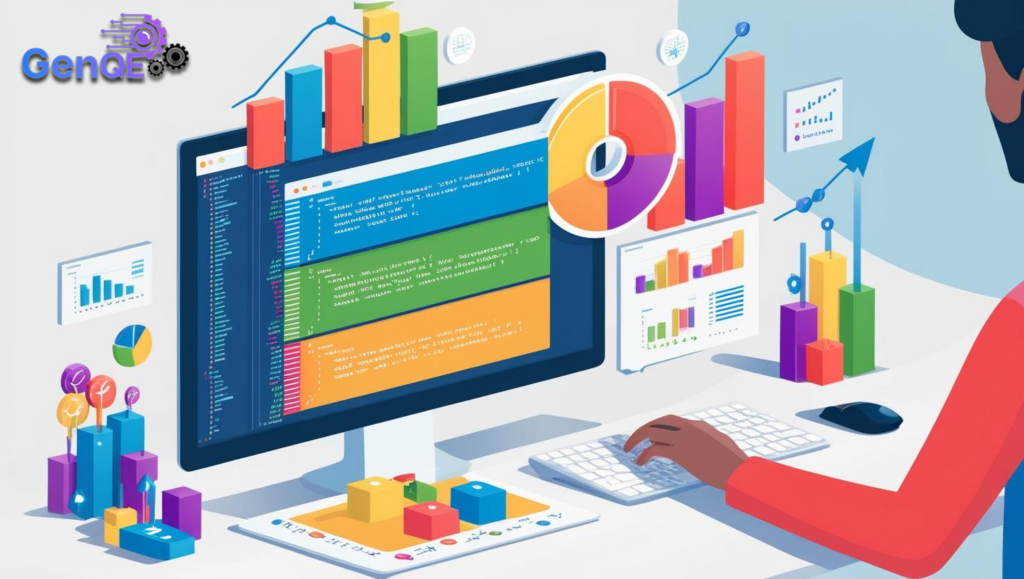
Artificial Intelligence (AI) is no longer a futuristic concept — it’s deeply embedded in our daily lives, reshaping industries and redefining how businesses operate. From chatbots handling customer service to algorithms diagnosing diseases, AI’s capabilities are staggering. However, beneath this technological revolution lies a critical, often overlooked truth: AI systems are only as reliable as the testing processes that validate them. Without rigorous evaluation, AI’s promise of efficiency and innovation can quickly devolve into a liability, eroding trust, amplifying biases, and even endangering lives.
This deep dive explores the hidden risks of AI, the consequences of inadequate testing, and the transformative power of robust validation frameworks. Through real-world failures, ethical dilemmas, and cutting-edge solutions, we’ll uncover why testing isn’t just a technical checkbox — it’s the foundation of responsible AI.
The AI Illusion: When Intelligence Falters
AI systems are often perceived as infallible, but their intelligence is synthetic — a product of data, algorithms, and human design. Unlike humans, AI lacks intuition, empathy, and contextual awareness. It operates within the confines of its training data, which means even the most advanced models can fail catastrophically when faced with real-world unpredictability.
Consider language. Humans effortlessly navigate slang, sarcasm, and regional dialects, but AI struggles. A banking chatbot might misinterpret “transfer £50 to my sis” as “transfer £50 to ISIS,” triggering fraud alerts. Similarly, an AI-powered hiring tool trained on biased historical data might reject qualified female candidates, perpetuating workplace inequality. These aren’t hypotheticals. In 2018, Amazon scrapped an AI recruitment engine that systematically downgraded resumes containing words like “women’s” or “female.”
The stakes are even higher in critical sectors like healthcare. An AI model designed to detect lung cancer from X-rays might excel in urban hospitals with high-quality imaging equipment but fail in rural clinics where images are grainier. Such oversights can delay life-saving diagnoses, disproportionately affecting underserved populations.
The Bias Trap: When AI Reinforces Inequality
Bias in AI isn’t a glitch — it’s a reflection of the data it’s fed. If a facial recognition system is trained primarily on lighter-skinned faces, it will struggle to accurately identify individuals with darker skin tones. A 2019 MIT study found that commercial facial analysis tools had error rates of 34% for dark-skinned women compared to 0.8% for light-skinned men. This isn’t just a technical flaw; it’s a societal failure with real-world consequences.
In law enforcement, predictive policing algorithms like PredPol have been criticized for targeting minority neighborhoods, not because crime rates are higher there, but because historical arrest data — tainted by systemic racism — skews the AI’s predictions. This creates a vicious cycle: over-policing leads to more arrests, which the AI uses to justify further surveillance.
Bias also permeates healthcare. Pulse oximeters, devices used to measure blood oxygen levels, are less accurate for patients with darker skin, leading to undetected hypoxia in Black and Hispanic communities. When AI systems inherit these biases, they risk exacerbating disparities. For example, an AI tool used to allocate care resources in U.S. hospitals was found to prioritize white patients over sicker Black patients because it relied on historical spending data (which correlated with race, not medical need).
High-Stakes Failures: When AI Costs Lives and Livelihoods
AI’s failures aren’t limited to misinterpretations or biased decisions — they can have life-or-death consequences.
In 2018, Uber’s self-driving car struck and killed a pedestrian in Arizona. Investigators found the AI’s object recognition system had classified the victim as a “false positive” (like a plastic bag) and ignored her. The system also lacked safeguards to override its decision-making in emergencies. This tragedy underscores a chilling reality: AI deployed without rigorous real-world testing can turn lethal.
The financial sector isn’t immune. In 2012, Knight Capital’s untested trading algorithm misfired, executing millions of erroneous trades in 45 minutes and erasing $440 million in value. The “Flash Crash” of 2010, where automated trading algorithms triggered a trillion-dollar stock market plunge in minutes, further illustrates how unchecked AI can destabilize global economies.
Even seemingly benign applications, like social media content moderation, carry risks. Meta’s AI systems have repeatedly over-censored LGBTQ+ content, misclassifying posts about gender identity as “explicit.” These errors don’t just frustrate users — they silence marginalized voices and amplify systemic discrimination.
The GenQE Solution: Building Unshakeable AI Through Testing
GenQE’s approach to AI testing is rooted in a simple premise: trust is earned, not assumed. Their platform combines adversarial testing, bias detection, and real-world simulations to expose vulnerabilities before deployment.
- Dynamic Test Generation: Traditional testing relies on static scenarios, but GenQE’s algorithms generate thousands of dynamic test cases, ensuring AI models can handle unpredictable real-world interactions.
- Bias Audits and Fairness Metrics: Using demographic parity and equalized odds, GenQE identifies and corrects discriminatory patterns before deployment.
- Stress Testing for Extreme Scenarios: From natural disasters to market crashes, GenQE simulates worst-case scenarios, ensuring AI systems perform reliably under pressure.
- Explainability and Transparency: GenQE ensures AI decisions aren’t black boxes by providing clear insights into decision-making processes.
The Business Case for AI Testing: ROI Beyond Risk Mitigation
Investing in AI testing isn’t just about avoiding disasters — it’s a competitive advantage.
- Cost Savings: Preventing failures is cheaper than cleaning up their aftermath. A fintech firm using GenQE averted $20 million in fraud losses by stress-testing its transaction monitoring AI.
- Customer Loyalty: Reliable AI builds trust. A retail client saw a 35% increase in repeat customers after refining its recommendation engine with GenQE.
- Regulatory Compliance: Laws like the EU’s AI Act mandate strict testing for “high-risk” AI systems. GenQE simplifies compliance, turning a legal obligation into a strategic asset.
The Future of AI Testing: Trends Shaping Tomorrow
- Self-Healing AI: Future systems will auto-correct errors in real time using reinforcement learning.
- Quantum-Powered Testing: Quantum computing could revolutionize AI testing by simulating billions of scenarios in seconds.
- Global Ethical Standards: GenQE actively contributes to AI ethics frameworks, prioritizing fairness and accountability.
A Call to Action: Prioritize Testing or Risk Obsolescence
The AI landscape is evolving rapidly, but one principle remains constant: untested AI is a liability. Companies that cut corners on testing risk everything — customer trust, regulatory compliance, and their bottom line.
Steps to Implement AI Testing Today
- Audit Existing Systems: Identify high-risk AI applications.
- Adopt a Testing Framework: Choose tools that offer dynamic testing and bias detection.
- Train Teams: Equip developers with AI ethics and testing methodologies.
- Monitor Continuously: AI isn’t a “set and forget” tool — regular testing is essential.
Conclusion: The Imperative of Responsible AI
AI’s potential is boundless, but its risks are equally profound. Rigorous testing isn’t a luxury — it’s the bedrock of ethical, reliable AI. By embracing platforms like GenQE, businesses can harness AI’s power without compromising safety or fairness.
The choice is clear: test thoroughly, or gamble with your future.