In the rapidly evolving world of software development, the stakes for maintaining high-quality standards have never been higher. Companies are under constant pressure to release flawless, innovative products that meet the ever-increasing expectations of users. Traditional software testing methodologies, while foundational, often fall short in keeping pace with the dynamic and complex requirements of modern applications. This blog post explores the intricate world of software testing, highlighting the challenges faced by QA teams and how leveraging AI-driven tools like GenQE can transform the testing landscape.
Software testing is no longer just a final step in the development process but a critical, ongoing activity that influences user satisfaction, system security, and the overall market success of a product. As we delve deeper, we will unravel the layers of software testing, introduce you to the next generation of testing tools, and demonstrate why integrating AI into your testing strategy isn’t just an option—it’s a necessity.
By the end of this article, you will gain a comprehensive understanding of the complexities of software testing, the inefficiencies of traditional methods, and how AI-powered solutions like GenQE are pioneering a shift towards more efficient, accurate, and cost-effective testing processes.
The Evolution of Software Testing
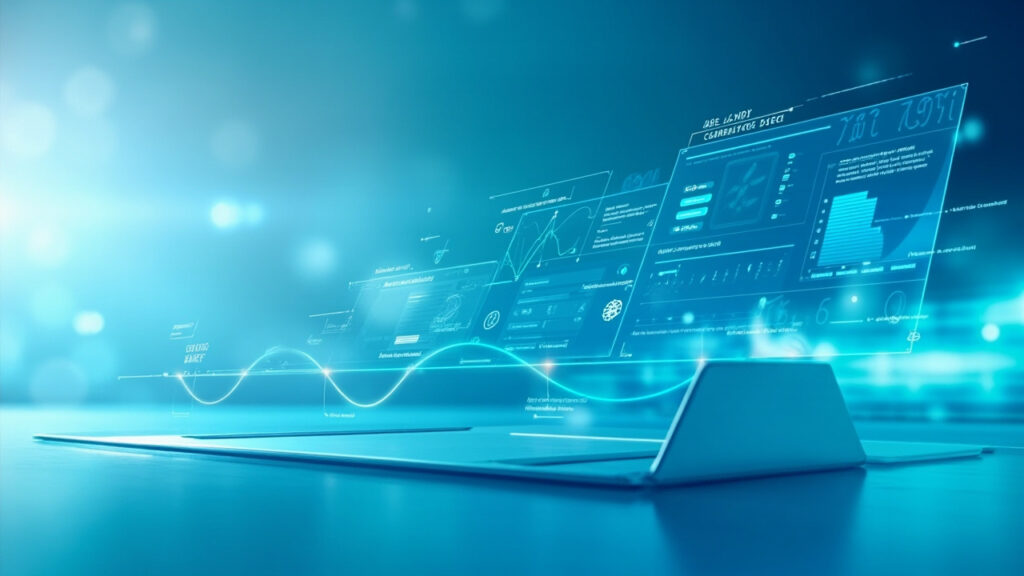
Software testing has undergone significant transformations over the decades, evolving from basic manual testing to more sophisticated, automated, and integrated approaches. This section will trace the milestones in the testing domain, highlight the advancements in methodologies, and discuss the pivotal role of technology in shaping modern testing practices.
Historical Perspective
In the early days of software development, testing was often performed ad-hoc, with limited tools and methodologies. Testers relied heavily on manual processes, making it time-consuming and prone to human error. As software systems grew in complexity, the need for more structured testing frameworks became evident.
Shift Towards Automation
The introduction of automated testing tools marked a significant shift in the landscape. Tools like Selenium and QTP (Quick Test Professional) allowed testers to automate repetitive tasks, leading to improvements in speed and reliability. However, despite these advancements, the rapidly changing requirements and environments posed new challenges.
Integration with Development Cycles
The adoption of Agile and DevOps practices further integrated testing into the development lifecycle. Continuous Integration (CI) and Continuous Deployment (CD) practices emphasized the need for ongoing testing, making it a continuous and integral part of development.
Challenges in Modern Software Testing
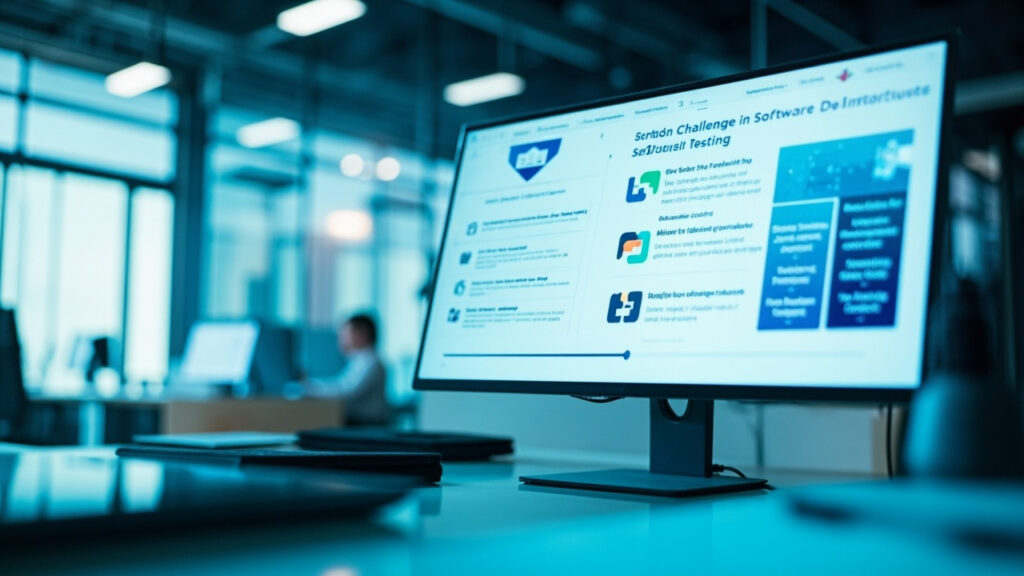
Despite advancements, several challenges persist in the realm of software testing. This section outlines the key obstacles that teams face today and sets the stage for understanding the need for more innovative solutions.
Keeping Pace with Development Speed
In the era of rapid development cycles, testing teams often struggle to keep pace with the continuous release of new features and updates. The traditional testing methods, which are time-consuming and labor-intensive, are frequently unable to meet these accelerated timelines.
Ensuring Comprehensive Coverage
Achieving complete testing coverage—where all aspects of the software are thoroughly tested—is increasingly difficult. The diversity of devices, platforms, and user environments adds layers of complexity to the testing process.
Dealing with Fluctuating Requirements
In dynamic development environments, requirements can change frequently. Adapting tests to these changes without introducing delays or errors is a significant challenge for traditional testing frameworks.
The Role of AI in Enhancing Software Testing
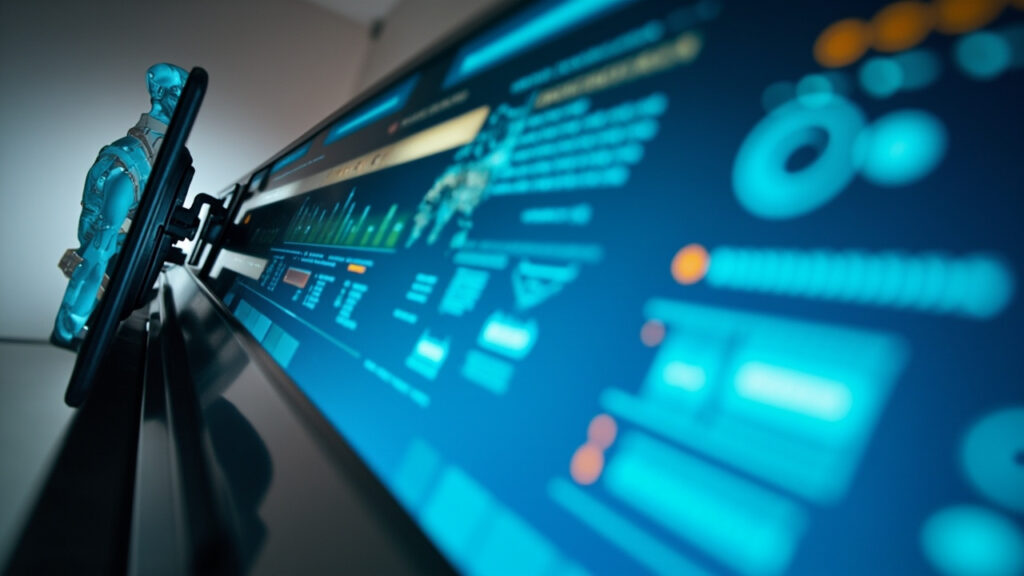
Artificial Intelligence (AI) is revolutionizing various industries, and software testing is no exception. AI can address many of the challenges faced by traditional methods, bringing about efficiency, accuracy, and scalability. Here, we will explore how AI is being integrated into testing processes and the benefits it offers.
AI-Driven Test Case Generation
One of the most time-consuming aspects of testing is the creation of test cases. AI can automate this process by analyzing application data, user interactions, and historical test results to generate relevant and comprehensive test cases.
Intelligent Test Execution
AI algorithms can prioritize test cases based on risk and potential impact, ensuring that critical areas of the application are tested first. This not only optimizes the testing efforts but also helps in early detection of major defects.
Enhancing Test Maintenance
AI-powered tools can automatically update test cases and scripts when there are changes in the application, significantly reducing maintenance effort and ensuring that tests remain relevant and effective.
Introducing GenQE: A Game-Changer in AI-Powered Testing
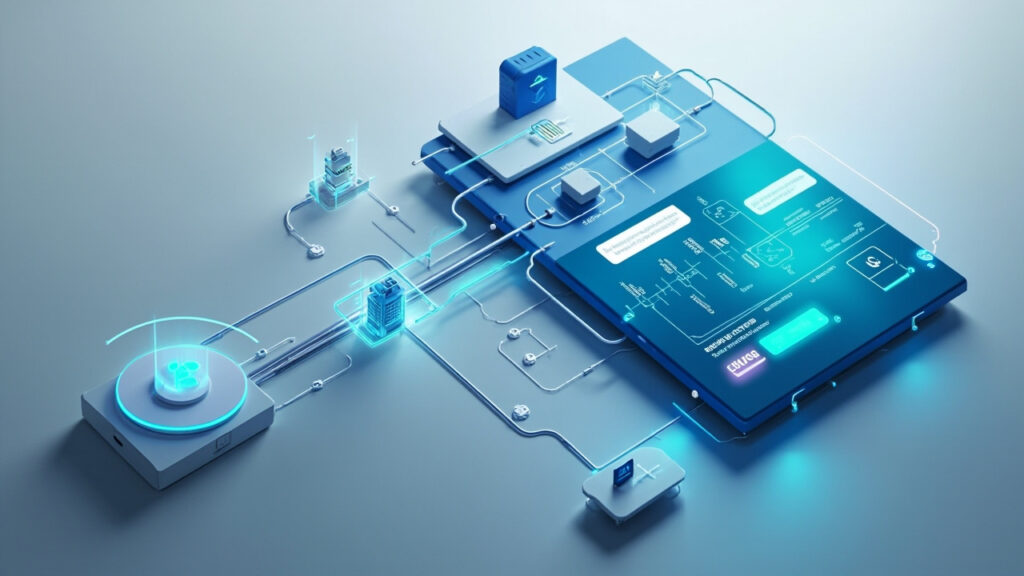
While discussing AI in software testing, it’s essential to spotlight tools like GenQE that epitomize the integration of AI technologies in quality assurance. GenQE stands out as a cutting-edge platform designed to enhance test automation, improve efficiency, and ensure robust software quality.
Key Features and Capabilities
- **AI-Driven Test Generation**: GenQE automates the creation and design of test cases, significantly reducing manual effort.
- **Smart Test Execution**: The platform intelligently analyzes risk to prioritize and execute test cases effectively.
- **Self-Healing Automation**: GenQE adapts to changes in the UI, maintaining the relevance and effectiveness of test scripts.
Strategic Integration
GenQE seamlessly integrates with popular DevOps tools, facilitating a smoother workflow and continuous testing environment. This integration is vital for teams adopting CI/CD practices, as it ensures that testing keeps pace with ongoing development changes.
Case Studies: Real-World Applications of AI in Testing
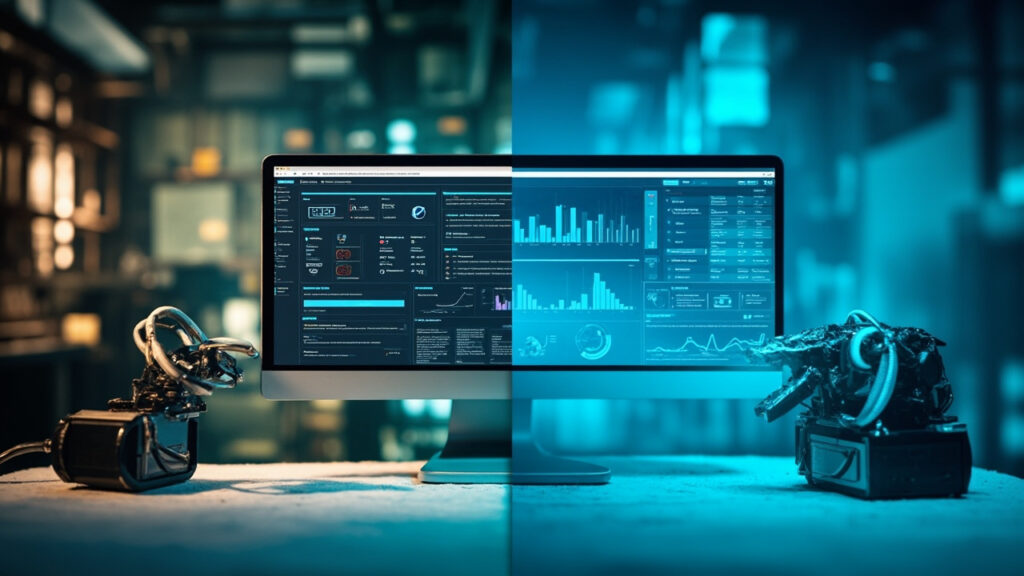
To illustrate the impact of AI in software testing, let’s examine a few case studies where AI-powered tools have significantly improved the testing outcomes.
E-commerce Platform Optimization
An e-commerce company implemented AI-driven testing to handle their vast array of products and complex user interfaces. The AI tool was able to quickly adapt to seasonal changes in the product lineup and user interface adjustments, reducing the testing cycle by 40% and increasing coverage by 25%.
Financial Software Compliance
A financial services provider used AI to enhance their testing processes to meet stringent compliance requirements. The AI tool not only expedited the testing process but also improved the accuracy of tests, ensuring compliance with industry standards.
Future Trends in Software Testing
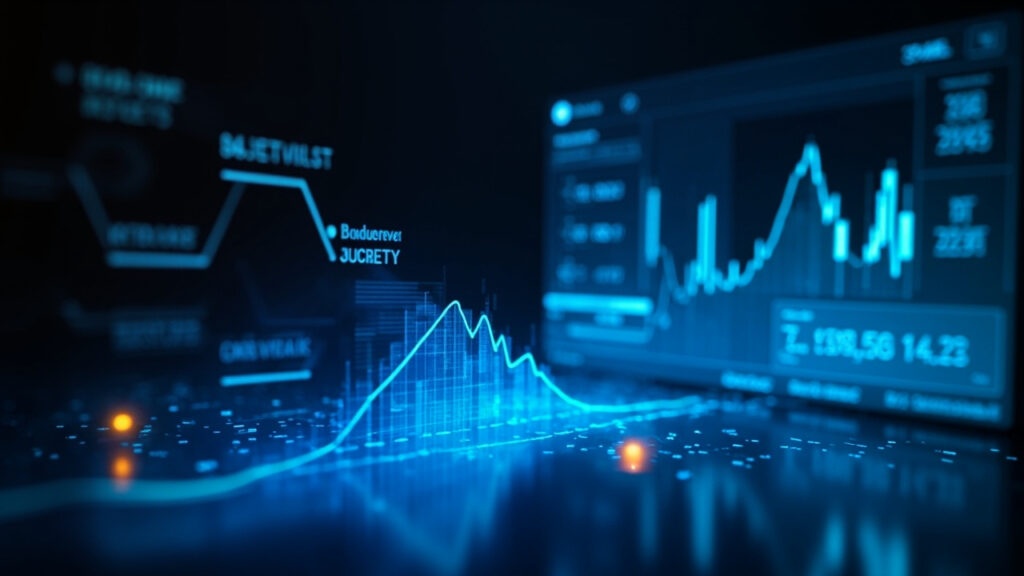
As we look to the future, several trends are set to shape the landscape of software testing. This section explores these trends and how they might influence testing strategies and tool development.
Predictive Analytics in Testing
The use of predictive analytics to foresee potential future defects and pinpoint areas of risk before they manifest is an exciting development. This proactive approach could dramatically reduce the incidence of bugs and enhance software reliability.
Increased Emphasis on Security Testing
With cybersecurity threats on the rise, there’s a growing need for more sophisticated security testing tools. AI can play a crucial role in identifying vulnerabilities and preventing security breaches before software is deployed.
User Experience-Driven Testing
As user expectations continue to evolve, testing that focuses on user experience (UX) will become increasingly important. AI can help simulate and test numerous real-world user scenarios, ensuring software not only works well but is also intuitive and user-friendly.
Conclusion: Embracing the Future with AI-Driven Testing
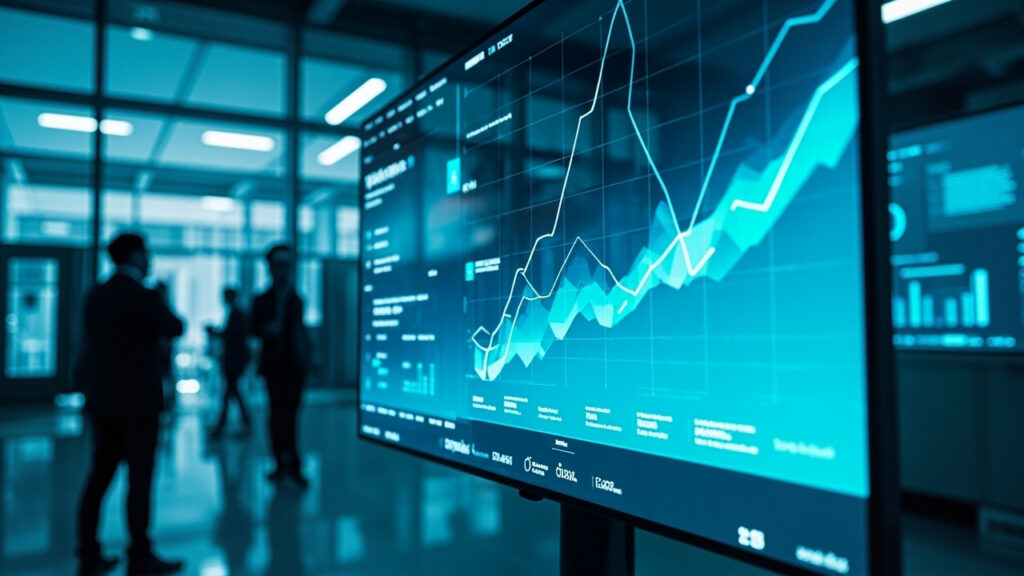
The landscape of software testing is shifting, with AI at the forefront of this transformation. For organizations aiming to enhance their quality assurance processes, reduce costs, and accelerate development cycles, adopting AI-driven tools like GenQE is not just beneficial—it’s imperative. As we have seen, the integration of AI in testing not only addresses current challenges but also sets the stage for more innovative, efficient, and secure software development practices.
In a world where software quality can make or break a business, staying ahead of the curve with advanced testing tools is not just a strategic advantage—it’s a necessity. If you’re looking to streamline your testing processes and embrace the future of software quality, exploring AI-powered solutions like GenQE could be your next step towards achieving higher efficiency and impeccable software quality.
Discover More Innovative Solutions
Want to learn more about the tools and technologies discussed in this article? Explore how these innovations can be tailored to your specific needs and workflow requirements.
Our team of experts is available to answer your questions and provide personalized insights into how modern solutions like GenQE can address your specific challenges.