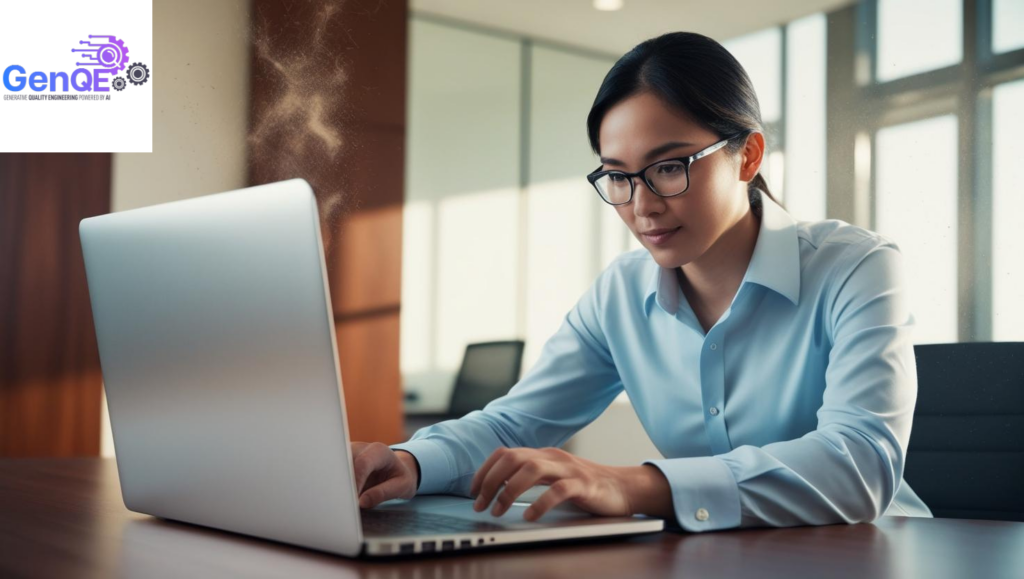
Artificial Intelligence (AI) is transforming industries by automating decision-making, improving efficiency, and enabling data-driven insights. However, as AI systems become more complex, the lack of transparency in their decision-making processes has raised concerns about bias, fairness, and accountability. This is where Explainable AI (XAI) plays a crucial role in building trustworthy and ethical AI systems.
Letโs explore the importance of Explainable AI, how it enhances trust, and its impact on real-world applications.
๐ 1๏ธโฃ What is Explainable AI (XAI)?
Explainable AI (XAI) refers to AI models and algorithms designed to provide clear, understandable, and interpretable explanations of their decisions and predictions. Unlike traditional “black-box” AI models, XAI ensures that stakeholdersโwhether end users, developers, or regulatorsโcan understand how and why an AI system reached a particular conclusion.
โ Key Goals of XAI:
- Increase transparency in AI-driven decisions
- Improve accountability and trust
- Detect and mitigate bias in AI models
- Ensure compliance with regulatory frameworks
๐ค 2๏ธโฃ Why is Explainability Critical for AI Adoption?
Trust is a fundamental requirement for AI adoption in critical industries such as healthcare, finance, law enforcement, and autonomous systems. When AI decisions impact human lives, organizations must ensure that these decisions are not only accurate but also understandable and justifiable.
โ How XAI Builds Trust:
- Improves User Confidence ๐: Users trust AI recommendations when they understand the reasoning behind them.
- Enhances Regulatory Compliance ๐: Governments and industry regulators demand AI systems that adhere to ethical guidelines (e.g., GDPR, AI Act).
- Reduces Bias and Discrimination โ๏ธ: Explainability helps identify and mitigate biases in AI models.
For example, an AI model rejecting a loan application without explaining why could lead to customer dissatisfaction and legal scrutiny. With XAI, financial institutions can justify their decisions and provide fair and transparent lending practices.
๐ฅ 3๏ธโฃ Real-World Applications of XAI
๐ 1. Healthcare: AI-Assisted Diagnosis
AI models are widely used in medical imaging, disease prediction, and drug discovery. However, doctors and patients need to understand AI-generated diagnoses to trust them. XAI ensures that AI-powered diagnoses are explainable, helping medical professionals make informed decisions.
โ Example: AI detects lung cancer in a scan but also provides reasons based on specific patterns and historical patient data.
๐ฐ 2. Finance: Fraud Detection & Credit Scoring
Banks and financial institutions use AI for fraud detection and credit risk assessment. If an AI system flags a transaction as fraudulent, XAI helps explain the decision, reducing false positives and customer frustration.
โ Example: Instead of simply declining a credit application, XAI explains factors like credit history, income level, and transaction behavior, ensuring fair decision-making.
๐ 3. Autonomous Vehicles: AI-Driven Decisions
Self-driving cars rely on AI to navigate, detect obstacles, and make real-time driving decisions. XAI helps developers and regulators understand why a vehicle made a specific maneuver, ensuring safety and reliability.
โ Example: If a self-driving car brakes suddenly, XAI can clarify whether it was due to a pedestrian crossing, road conditions, or an object on the road.
๐ 4๏ธโฃ Regulatory and Ethical Considerations
Governments and organizations worldwide are implementing regulations to ensure AI systems are transparent and accountable.
โ Key AI Regulations Promoting Explainability:
- GDPR (General Data Protection Regulation, EU): Requires AI-based decisions to be explainable, especially in cases affecting human rights.
- EU AI Act: Proposes risk-based AI regulations emphasizing transparency and fairness.
- US AI Bill of Rights: Focuses on ensuring AI systems are ethical and explainable.
Businesses adopting AI must prioritize explainability to comply with these regulations and avoid legal risks.
๐ 5๏ธโฃ The Future of Explainable AI
As AI adoption accelerates, the demand for human-centric AI will grow. Future advancements in XAI will focus on:
๐น Better Visualization Techniques ๐ โ Interactive dashboards and heatmaps to interpret AI decisions.
๐น AI Model Transparency Standards ๐ โ Industry-wide guidelines for AI explainability.
๐น Human-AI Collaboration ๐ค โ AI systems that work alongside humans, providing real-time justifications.
Businesses investing in AI should integrate XAI from the ground up to ensure their AI models are fair, interpretable, and aligned with human values.
๐น Conclusion: Trustworthy AI is Explainable AI
Explainable AI is no longer optionalโit is a necessity for organizations aiming to build trustworthy AI systems. By making AI decisions transparent, interpretable, and accountable, businesses can gain user confidence, comply with regulations, and drive ethical AI adoption.
๐ As AI continues to shape the future, organizations that prioritize explainability will lead the way in responsible AI innovation.
๐น Do you think Explainable AI should be mandatory in all industries? Letโs discuss in the comments! ๐ฌ