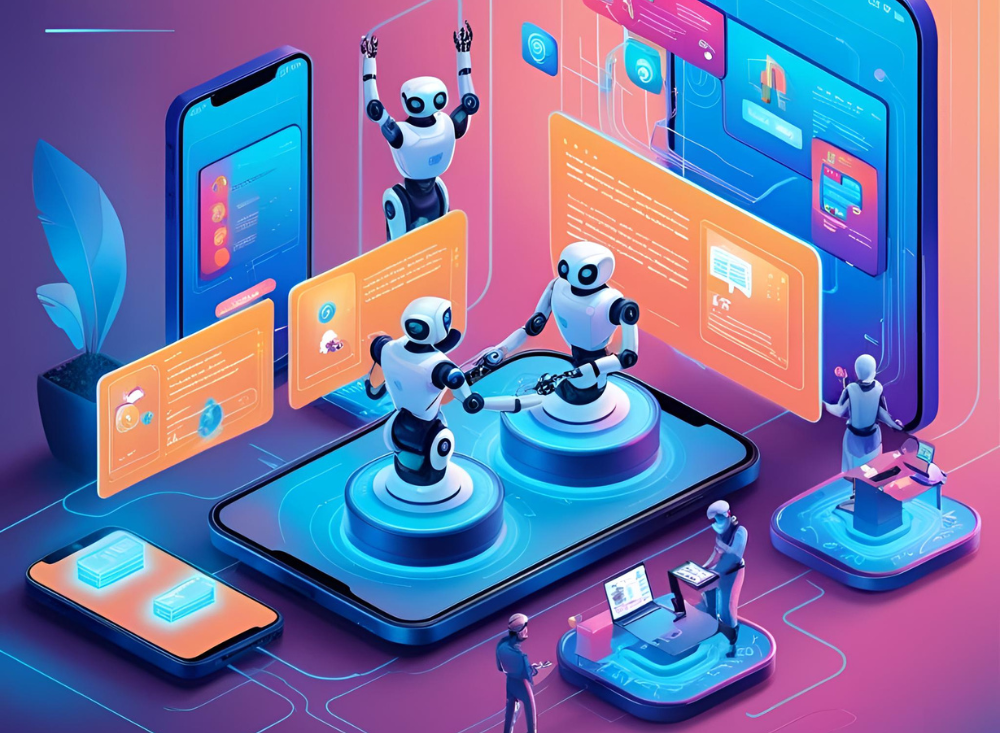
Introduction: The AI Testing Revolution
Mobile app testing is undergoing a radical transformation as artificial intelligence reshapes traditional quality assurance approaches. By 2025, over 60% of enterprises will incorporate AI-driven testing solutions into their mobile development pipelines, according to recent industry forecasts. This shift comes as apps grow more complex while release cycles accelerate beyond human testing capabilities.
How AI is Transforming Mobile Testing
1.Intelligent Test Case Generation
Modern AI algorithms now analyze:
- User behavior patterns
- Historical defect data
- Code change impact
To automatically generate optimized test scenarios that maximize coverage while minimizing redundancy. Unlike static test scripts, these AI-generated cases evolve with the application.
2.Self-Healing Test Automation
Leading solutions now feature:
- Dynamic element locator adaptation
- Context-aware script repair
- Automatic test flow adjustments
Reducing maintenance overhead by up to 70% compared to traditional automation frameworks. Tests automatically adjust to UI changes without human intervention.
3.Visual Validation at Scale
Computer vision enables:
- Pixel-perfect layout testing
- Cross-device rendering verification
- Dynamic content validation
Across thousands of device configurations simultaneously, something impossible with manual testing.
4.Predictive Analytics for Quality
Machine learning models now:
- Forecast defect-prone areas
- Recommend test prioritization
- Predict release readiness
Based on historical data patterns, helping teams focus testing efforts where they matter most.
The Business Impact of AI Testing
1.Accelerated Release Velocity
Early adopters report:
- 40–60% faster test cycles
- 80% reduction in escaped defects
- 3–5x more frequent releases
Without compromising quality standards.
2.Enhanced Test Coverage
AI-driven testing achieves:
- 95%+ path coverage
- Real-world scenario simulation
- Edge case discovery
That manual test planning often misses.
3.Cost Optimization
Organizations realize:
- 30–50% lower testing costs
- Reduced device lab expenses
- Minimal test maintenance
Through intelligent automation.
Implementation Challenges
1.Skills Gap
Teams must develop:
- AI test strategy expertise
- Model training capabilities
- Results interpretation skills
To fully leverage these technologies.
2.Data Quality Requirements
Effective AI testing demands:
- Comprehensive historical data
- Well-labeled defect reports
- Clean test execution records
For accurate model training.
3.Change Management
Successful adoption requires:
- Process re-engineering
- Role redefinition
- Cultural adaptation
Across QA organizations.
The Future of AI in Mobile Testing
Emerging innovations include:
- Autonomous testing agents that self-improve
- Natural language test case generation
- Real-time production monitoring with auto-remediation
- Blockchain-verified test results
Conclusion: The AI Testing Imperative
As mobile apps become more sophisticated and user expectations rise, AI-powered testing transitions from competitive advantage to necessity. Platforms like Genqe.ai demonstrate how intelligent automation can deliver superior quality at unprecedented speed. Organizations that embrace these technologies today will lead their markets tomorrow, turning quality assurance from a bottleneck into a strategic differentiator.
The question is no longer whether to adopt AI in mobile testing, but how quickly and effectively organizations can implement these transformative capabilities across their development lifecycle.