In the ever-evolving world of software development, ensuring the quality of applications is more critical than ever. As developers race to deliver new features and updates, the pressure on software testing teams intensifies. Traditional testing methods, though foundational, often struggle to keep pace with the rapid development cycles and increasing complexity of modern applications. This article delves into the intricacies of software testing, exploring its challenges, methodologies, and the innovative tools that are transforming the landscape.
With the stakes so high, how can teams ensure software quality without compromising on speed or efficiency? This post will unravel the complexities of software testing, offering insights into emerging technologies, including AI-powered solutions like GenQE, and practical strategies to enhance testing processes.
The Evolution of Software Testing: From Manual to Automated
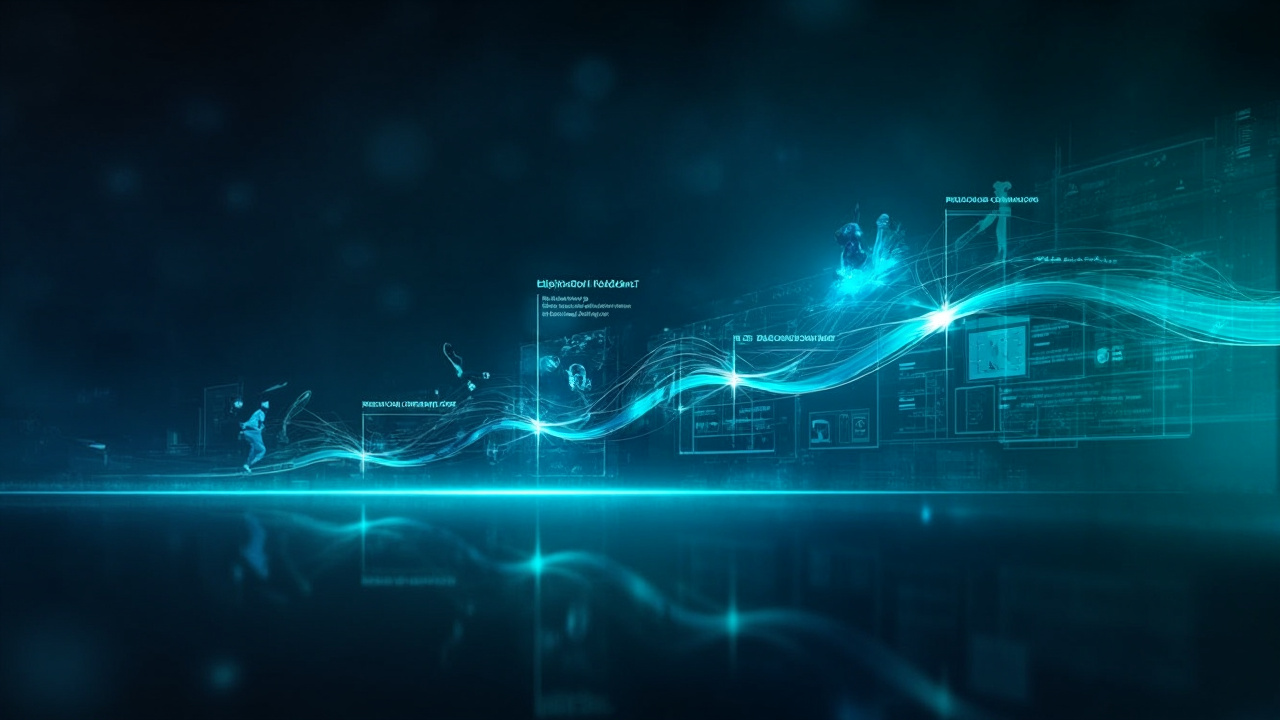
Software testing has undergone a significant transformation over the decades. Initially, testing was a labor-intensive, manual process, involving meticulous checks and balances to ensure software functionality. As software projects grew in complexity, the limitations of manual testing became apparent. Enter automation a game-changer that promised to speed up the testing process and improve accuracy.
The Rise of Automated Testing
Automated testing introduced the ability to run repetitive tests quickly, freeing testers from mundane tasks and allowing them to focus on more complex testing scenarios. This shift increased the efficiency of testing teams and reduced human error, providing a more reliable measure of software quality. For instance, regression testing, which is critical for verifying that new code changes do not adversely affect existing functionalities, can be executed swiftly and repeatedly with automation.
“Automation is not about replacing testers; it’s about empowering them to do more.”
However, automation is not without its challenges. Maintenance of test scripts can be cumbersome, especially when dealing with frequently changing software elements. For example, a minor change in the user interface can render a test script obsolete, requiring updates that consume time and resources. This has led to the development of more sophisticated tools that incorporate self-healing capabilities to address these issues. GenQE, for instance, offers self-healing automation that adapts to UI changes automatically, ensuring that test scripts remain functional without constant manual intervention.
Bridging the Gap with AI
Artificial intelligence is the latest frontier in software testing. AI-driven tools like GenQE are enhancing automation by introducing capabilities such as intelligent test generation and smart execution. These tools analyze vast amounts of data to optimize testing processes, ensuring comprehensive coverage and efficient defect detection. By leveraging machine learning algorithms, GenQE can predict which areas of the software are most likely to contain defects, allowing testers to prioritize these areas and focus their efforts more effectively.
The integration of AI into automated testing is not just an evolutionary step but a revolutionary leap, offering unprecedented levels of accuracy and efficiency. For example, AI can simulate user behavior more realistically, ensuring that tests reflect real-world usage patterns and uncover potential issues that traditional testing might miss.
Understanding the Software Testing Lifecycle
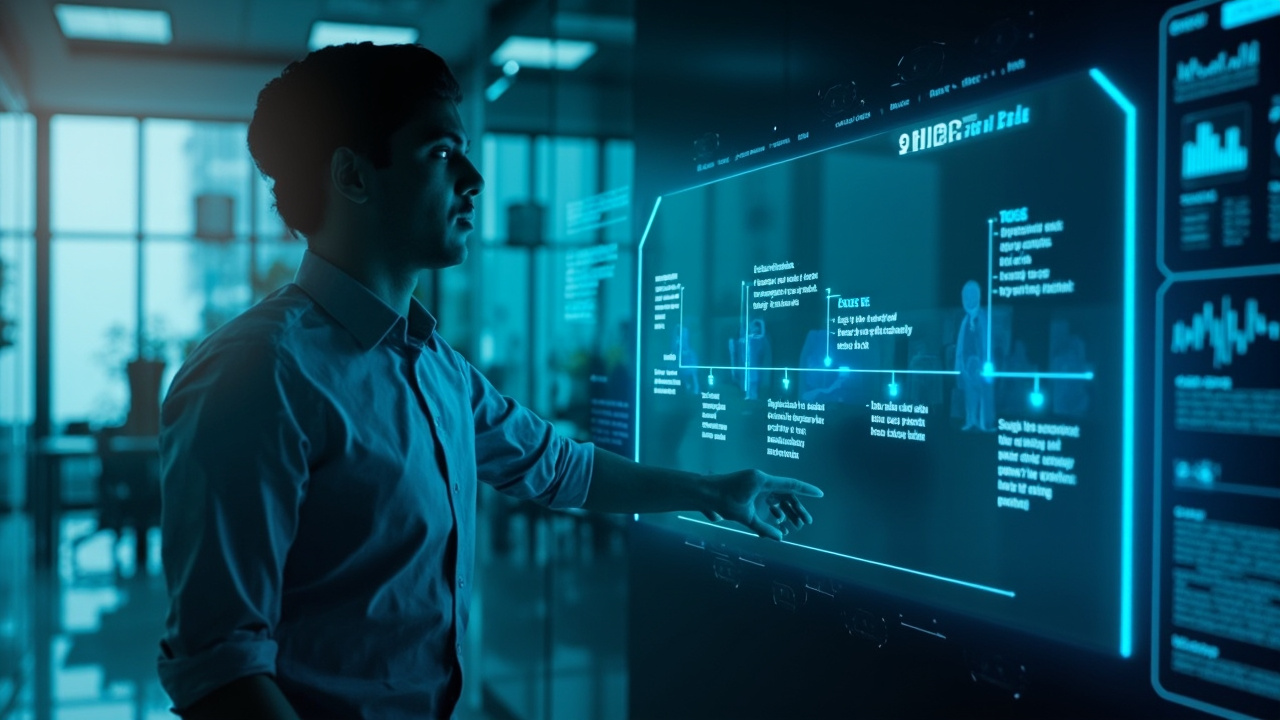
To appreciate the full potential of modern testing tools, it’s crucial to understand the software testing lifecycle. This structured process ensures that every aspect of the software is thoroughly evaluated before release.
Key Phases of Testing
- Requirement Analysis: This initial phase involves understanding the software’s functionality and performance requirements. Testers collaborate with stakeholders to gather detailed requirements that inform the testing strategy. For example, understanding user needs and regulatory requirements can help create test cases that ensure compliance and user satisfaction.
- Test Planning: Here, the scope of testing is defined. Test plans and strategies are developed, specifying the resources and timelines required to achieve testing goals. A well-crafted test plan outlines the objectives, resources, schedule, and deliverables, acting as a roadmap for the testing process.
- Test Case Development: Test cases are designed based on the requirements. This involves creating detailed scenarios that cover all possible user interactions with the software. Effective test cases are clear, concise, and cover both positive and negative scenarios to ensure comprehensive testing.
- Test Environment Setup: A critical phase where the testing environment is configured. This includes setting up hardware and software resources necessary for executing test cases. An accurate test environment mirrors the production environment, ensuring that test results are reliable and relevant.
- Test Execution: This is where the rubber meets the road. Test cases are executed, and outcomes are recorded. Any deviations from expected results are logged as defects. Test execution is iterative, with each cycle refining the software and addressing identified issues.
- Test Closure: After testing, results are evaluated against the initial requirements. A test closure report is generated, summarizing the findings and providing insights for future projects. This phase involves documenting lessons learned and assessing the quality of the testing process.
“A well-defined testing lifecycle is the backbone of successful software quality assurance.”
Enhancing the Lifecycle with AI
AI tools like GenQE enhance the testing lifecycle by introducing automation and intelligence at every stage. From generating test cases to executing them intelligently, GenQE ensures that testing is not only comprehensive but also efficient. For instance, during test execution, GenQE can dynamically adjust test priorities based on real-time analysis, ensuring that critical issues are addressed promptly.
By integrating AI into the testing lifecycle, teams can reduce the time and effort required for testing while improving the overall quality of the software. This approach allows organizations to deliver high-quality software faster, meeting the demands of today’s competitive market.
Challenges in Modern Software Testing
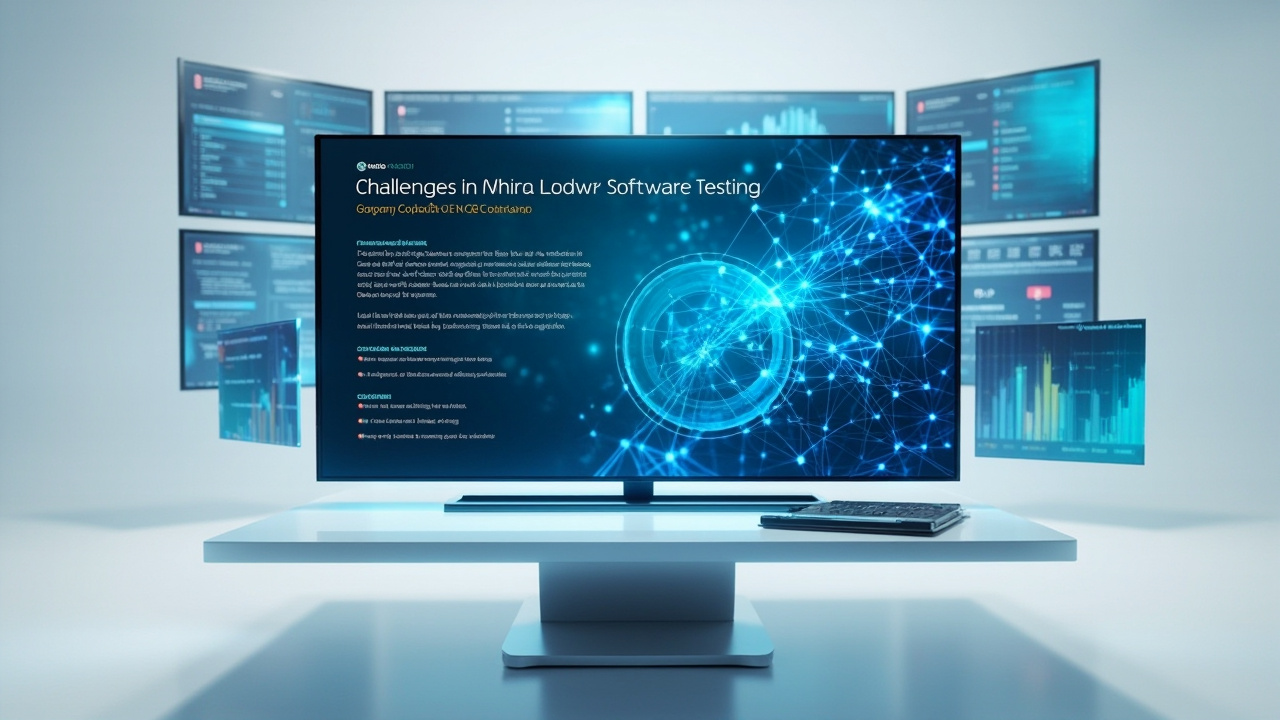
Despite advancements, software testing teams face numerous challenges. These challenges arise from the complexity of applications, the need for rapid deployment, and the ever-present pressure to reduce costs.
Common Testing Challenges
- Complexity and Scale: Modern applications are more complex, often spanning multiple platforms and devices. This complexity increases the testing workload significantly. For example, a banking application must function seamlessly across web, mobile, and ATM interfaces, each with unique testing requirements.
- Time Constraints: With rapid deployment cycles, testing teams have limited time to ensure comprehensive coverage, increasing the risk of defects slipping through. Agile methodologies emphasize speed, often squeezing the testing window and necessitating efficient, automated solutions.
- Resource Limitations: Testing often competes with other project priorities for resources, making it difficult to allocate sufficient time and manpower to testing activities. This can lead to inadequate testing and increased risk of defects in production.
- Maintenance Overhead: Automation scripts require constant updates to remain effective, consuming valuable resources that could be used for actual testing. For instance, a script designed to test a login function may need frequent updates if the login process or UI changes regularly.
Innovative Solutions
To address these challenges, teams are turning to innovative solutions. AI-powered platforms like GenQE offer features such as self-healing automation and risk-based test execution, which significantly reduce maintenance overhead and optimize testing efforts. By prioritizing tests based on risk, GenQE ensures that the most critical parts of the application are tested first, reducing the likelihood of high-impact defects.
“In the face of complexity, innovation is not just an advantage; it’s a necessity.”
By leveraging these tools, teams can overcome resource constraints and deliver high-quality software without compromising on speed or efficiency. AI-driven insights provide real-time feedback, allowing teams to make informed decisions and adjust their strategies as needed.
Best Practices for Effective Software Testing
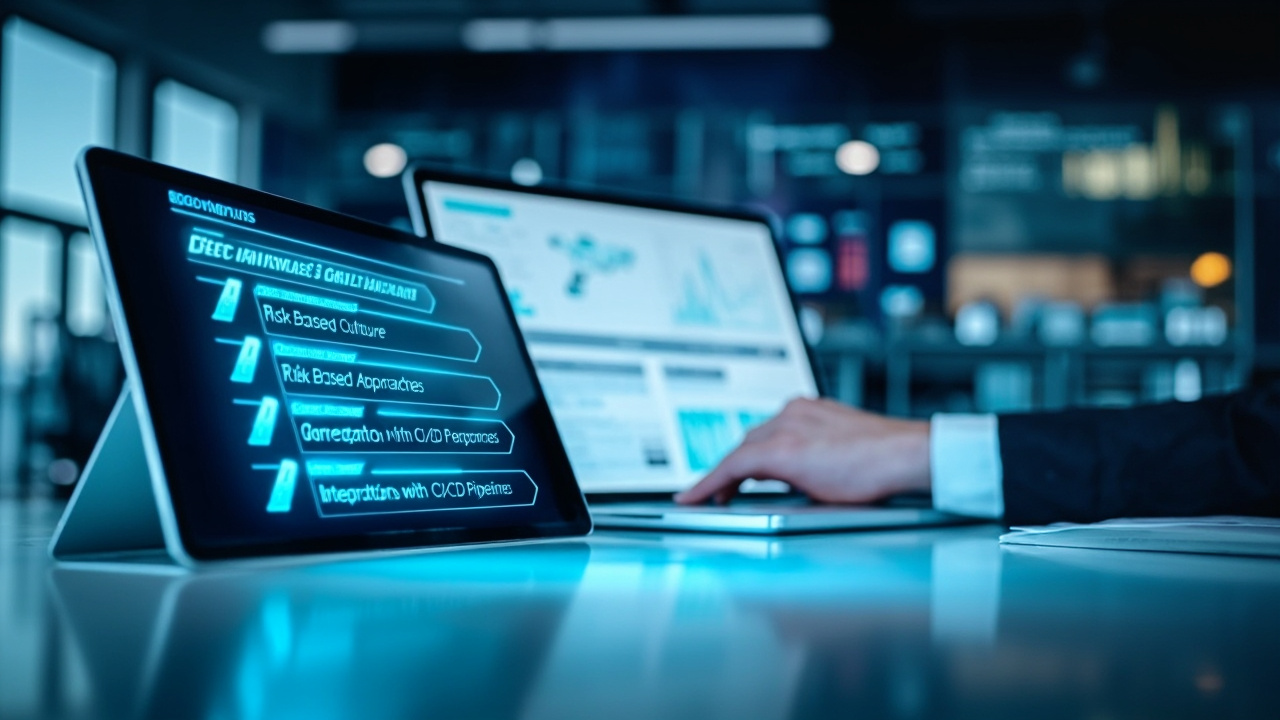
While tools and technologies are essential, the foundation of effective software testing lies in best practices. These practices ensure that testing is thorough, efficient, and aligned with overall project goals.
Establishing a Testing Culture
Creating a culture that values testing is crucial. Teams should view testing as an integral part of the development process rather than an afterthought. This mindset ensures that quality is prioritized from the outset. Encouraging collaboration between developers and testers fosters a shared responsibility for quality and promotes a proactive approach to testing.
Adopting a Risk-Based Approach
Not all software components require the same level of testing. By adopting a risk-based approach, teams can prioritize testing efforts on high-impact areas, ensuring that critical functionalities are thoroughly evaluated. This approach involves assessing the likelihood and impact of potential defects and allocating resources accordingly. For example, a payment processing system in an e-commerce application would warrant more rigorous testing than a non-essential feature.
Continuous Integration and Delivery
Integrating testing into continuous integration and delivery (CI/CD) pipelines ensures that testing occurs throughout the development cycle. This approach provides immediate feedback on software quality and accelerates release cycles. Automated tests are triggered with each code commit, ensuring that defects are identified and addressed early in the development process.
Leveraging AI and Automation
Incorporating AI and automation can transform testing processes. Tools like GenQE offer automated test generation and intelligent execution, reducing the manual effort required and enhancing overall efficiency. By analyzing historical data and user behavior, AI tools can generate test cases that are more likely to uncover defects, ensuring comprehensive coverage.
“Testing is not a phase; it’s a mindset.”
These best practices, when implemented effectively, can significantly enhance the quality and reliability of software products. A commitment to continuous improvement and adaptation is key to maintaining high standards of quality in a rapidly changing software landscape.
The Role of AI in Transforming Software Testing
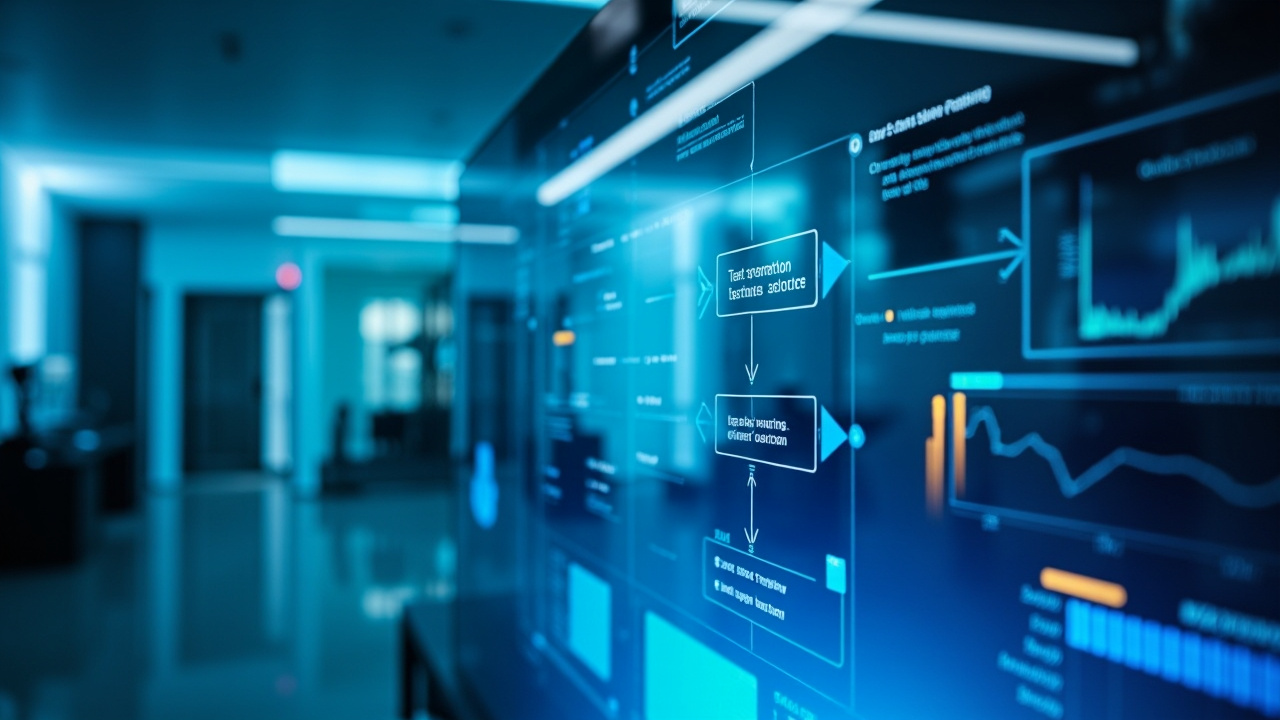
Artificial intelligence is reshaping software testing, offering new levels of efficiency and accuracy. But what exactly can AI bring to the table, and how can it be integrated into existing testing frameworks?
AI-Driven Test Generation
AI tools analyze vast amounts of data, including software requirements and historical test results, to automatically generate test cases. This reduces the dependency on manual test case design and ensures comprehensive test coverage. AI can identify patterns and trends that human testers might overlook, generating test scenarios that more accurately reflect real-world usage.
Smart Test Execution
By prioritizing test cases based on risk analysis, AI-driven tools ensure that critical areas are tested first. This strategic approach optimizes testing efforts and focuses resources on high-impact defects. For instance, GenQE’s smart execution capabilities allow testing teams to concentrate on areas with the highest potential for defects, improving overall test efficiency.
“AI doesn’t replace the human element in testing; it enhances it.”
Self-Healing Automation
Traditional test scripts often break when UI elements change. AI-powered self-healing capabilities automatically adjust test scripts, minimizing maintenance efforts and ensuring continuous test execution. This feature is particularly valuable in agile environments, where frequent changes to the codebase are common.
Enhanced Defect Detection
AI tools like GenQE use machine learning to identify patterns in test results, detecting anomalies and potential defects early in the development cycle. This proactive approach prevents critical issues from reaching production. By continuously analyzing test data, AI can provide insights into the root causes of defects, enabling teams to address underlying issues rather than just symptoms.
AI’s integration into software testing is not just a trend but a necessity. As software complexity continues to grow, AI offers the only feasible path to maintaining high standards of quality without sacrificing speed. By automating repetitive tasks and providing deep insights, AI empowers testers to focus on strategic testing activities that add the most value.
Case Studies: AI in Action
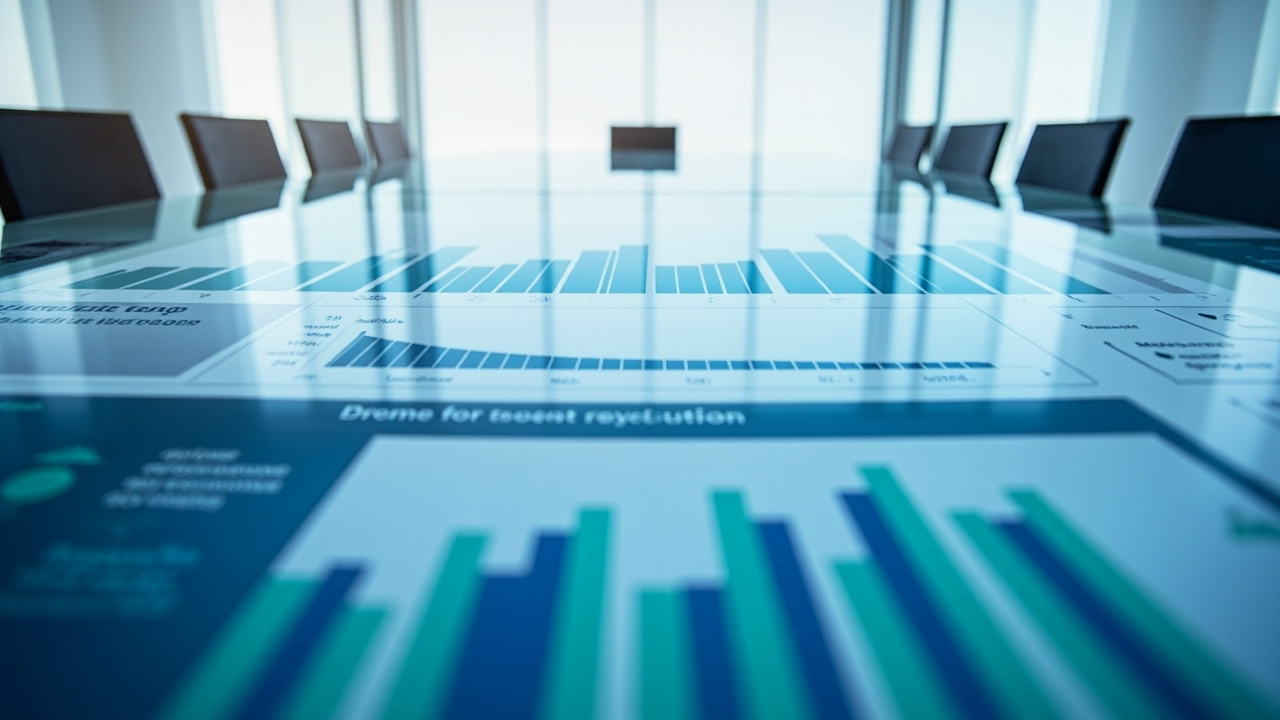
To understand the impact of AI on software testing, let’s explore some real-world examples of how organizations have leveraged AI tools to enhance their testing processes.
Case Study 1: Enhancing Test Coverage
A leading e-commerce company faced challenges with test coverage due to the complexity of its platform. By integrating GenQE, they automated test case generation, resulting in a 30% increase in test coverage and a 40% reduction in testing time. The AI-driven approach allowed them to quickly adapt to changing requirements and maintain high standards of quality across multiple releases.
Case Study 2: Reducing Maintenance Overhead
A financial services firm struggled with the high maintenance costs of test scripts. GenQE’s self-healing automation reduced maintenance efforts by 50%, allowing the team to focus on developing new test scenarios. This not only improved efficiency but also enhanced the team’s ability to respond to business needs rapidly.
“Real-world success stories highlight the transformative power of AI in software testing.”
Case Study 3: Improving Defect Detection
A healthcare company aiming to improve software reliability integrated AI-based defect detection. The proactive identification of defects reduced production issues by 60%, significantly enhancing software quality. The AI system continuously learned from testing results, refining its ability to detect subtle defects and improving overall system reliability.
These case studies demonstrate the tangible benefits of incorporating AI tools like GenQE into software testing processes, offering inspiration for organizations seeking to enhance their testing frameworks. By leveraging AI, these companies achieved greater efficiency, reduced costs, and improved software quality, positioning themselves for success in a competitive market.
Conclusion: Embracing the Future of Software Testing
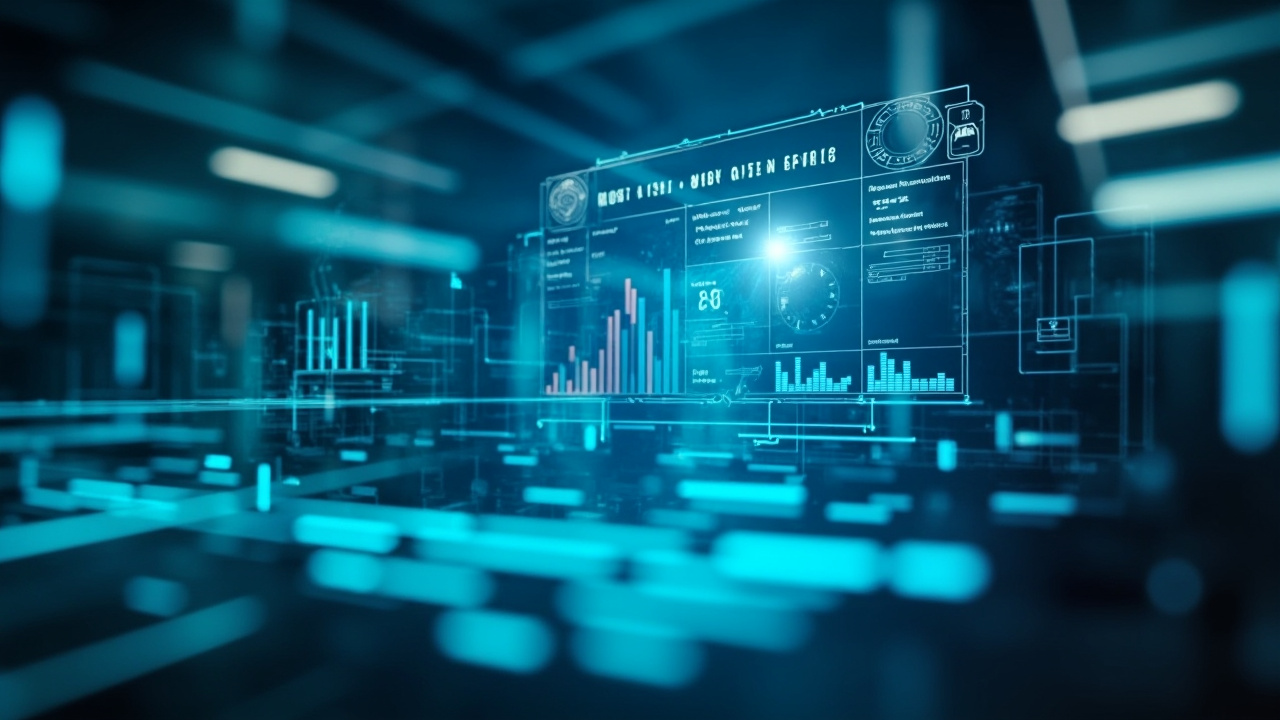
As the software development landscape continues to evolve, so too must our approaches to testing. The integration of AI into software testing is no longer optional; it is essential for maintaining quality in an increasingly complex and fast-paced environment.
By adopting innovative tools like GenQE and incorporating best practices, organizations can enhance their testing processes, reduce costs, and accelerate time to market. The future of software testing is here, and it holds the promise of more reliable, efficient, and intelligent testing methodologies.
For those looking to stay ahead of the curve, exploring AI-powered solutions like GenQE offers an opportunity to revolutionize testing processes and ensure the highest standards of software quality. By embracing these technologies, organizations can not only keep pace with industry changes but also set new benchmarks in software excellence.
“The future of software testing is intelligent, adaptive, and relentless in its pursuit of quality.”
In conclusion, as we stand on the brink of a new era in software testing, the call to action is clear: embrace innovation, leverage the power of AI, and transform your testing processes to meet the demands of tomorrow’s software landscape. By doing so, organizations can deliver exceptional software that meets and exceeds user expectations, driving success and growth in an ever-competitive market.
Discover More Innovative Solutions
Want to learn more about the tools and technologies discussed in this article? Explore how these innovations can be tailored to your specific needs and workflow requirements.
Our team of experts is available to answer your questions and provide personalized insights into how modern solutions like GenQE can address your specific challenges.
If the link above does not work, please visit: https://calendly.com/dm-csimplifyit/30min?month=2025-05