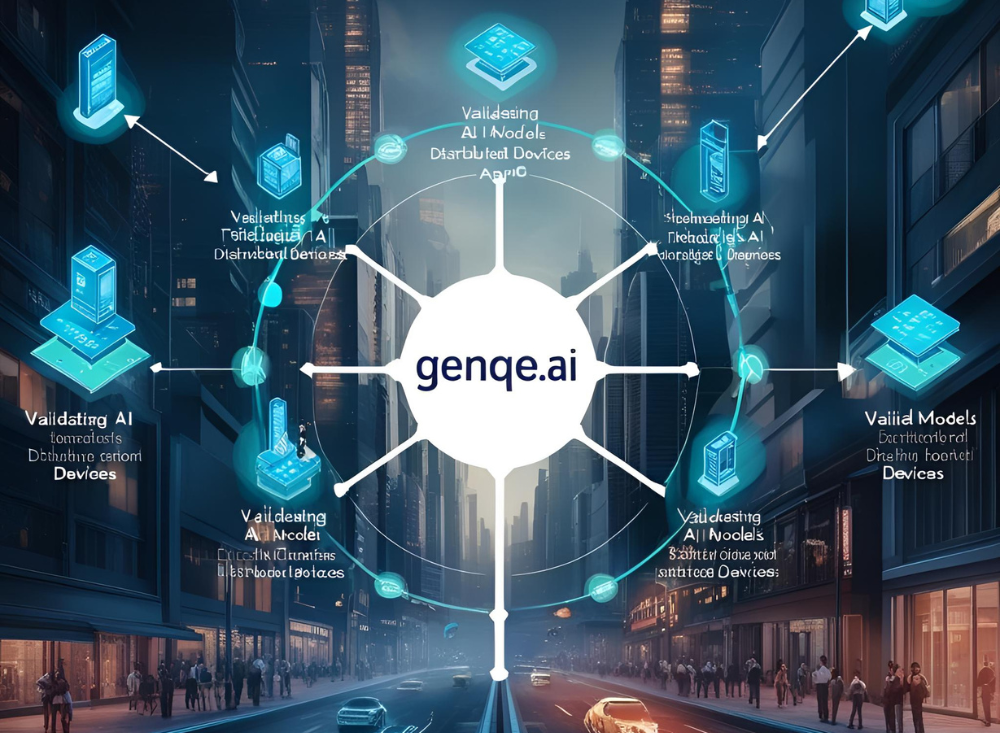
As AI adoption accelerates across industries, the infrastructure supporting intelligent applications is evolving just as quickly. Federated learning — where AI models are trained and operated across distributed devices like mobile phones, wearables, and IoT sensors — has emerged as a game-changing approach for privacy-preserving AI. But with it comes an important and complex question: how do you test these models effectively when your system spans across hundreds or even thousands of unique environments?
This is where federated app testing comes into play — and in 2025, testing tools must keep pace with this distributed reality. Enter Genqe.ai, an AI-powered testing platform built for the modern, decentralized ecosystem.
The Challenge of Testing AI in a Federated World
Traditional testing practices operate under the assumption that the environment is controlled, data is centralized, and deployments are relatively uniform. Federated learning flips that on its head. It decentralizes both the data and the model training, making it harder to predict behavior across devices. You’re not just testing one version of an app anymore — you’re validating how the model performs across thousands of data environments, network conditions, hardware capabilities, and even regional user behaviors.
If your testing process doesn’t reflect that complexity, you’re likely missing hidden bugs, biases, and performance issues that only surface at the edge.
How Genqe.ai Addresses the Testing Gap
Genqe.ai offers a tailored solution for this exact scenario — an intelligent platform that understands how to test federated applications by leveraging the power of AI itself. Here’s how it transforms the federated app testing process:
1. Automated Test Generation for Device Variability
Genqe.ai uses AI algorithms to generate test scenarios that reflect real-world variations in devices and conditions. Instead of creating one-size-fits-all test cases, it builds a portfolio of test scenarios tuned for multiple device profiles — from high-end smartphones to low-power edge devices.
2. Behavioral Monitoring at Scale
Once deployed, the platform continuously monitors how models behave across distributed nodes. If a model behaves differently on Device A vs Device B, Genqe.ai detects that anomaly automatically and flags it. This real-time visibility is key in identifying performance bottlenecks or logic errors early.
3. Built-in Bias and Fairness Validation
Federated environments often reflect diverse user populations — which means AI models are at risk of learning unintentional biases. Genqe.ai runs automated audits to evaluate fairness across demographic groups and devices, helping developers catch and fix discriminatory behavior before it impacts users.
4. Insightful Analytics and Explainability
Genqe.ai not only flags what’s wrong — it explains why. With built-in explainability features, engineers get actionable insights into how the model is making decisions across devices. That transparency leads to faster root-cause analysis and more confident releases.
Why Federated App Testing Matters in 2025
Testing AI models across distributed environments isn’t just a technical checkbox — it’s a necessity. With the rise of super apps, edge computing, and embedded intelligence in consumer electronics, federated testing ensures:
- Consistency: AI models behave the same way no matter the environment.
- Compliance: You stay ahead of privacy regulations and ethical AI guidelines.
- Reliability: End users get a seamless experience, regardless of device or location.
- Scalability: Teams can confidently release new updates to federated networks without fearing mass failures.
Conclusion: Test Smarter with Genqe.ai
As federated AI becomes the new normal, legacy testing tools simply can’t keep up. Genqe.ai is engineered for this next chapter — helping teams validate, monitor, and optimize AI models across the complex, distributed ecosystems of 2025 and beyond.
Whether you’re building for wearables, autonomous systems, or decentralized apps, Genqe.ai is your AI-powered partner in delivering quality at scale.