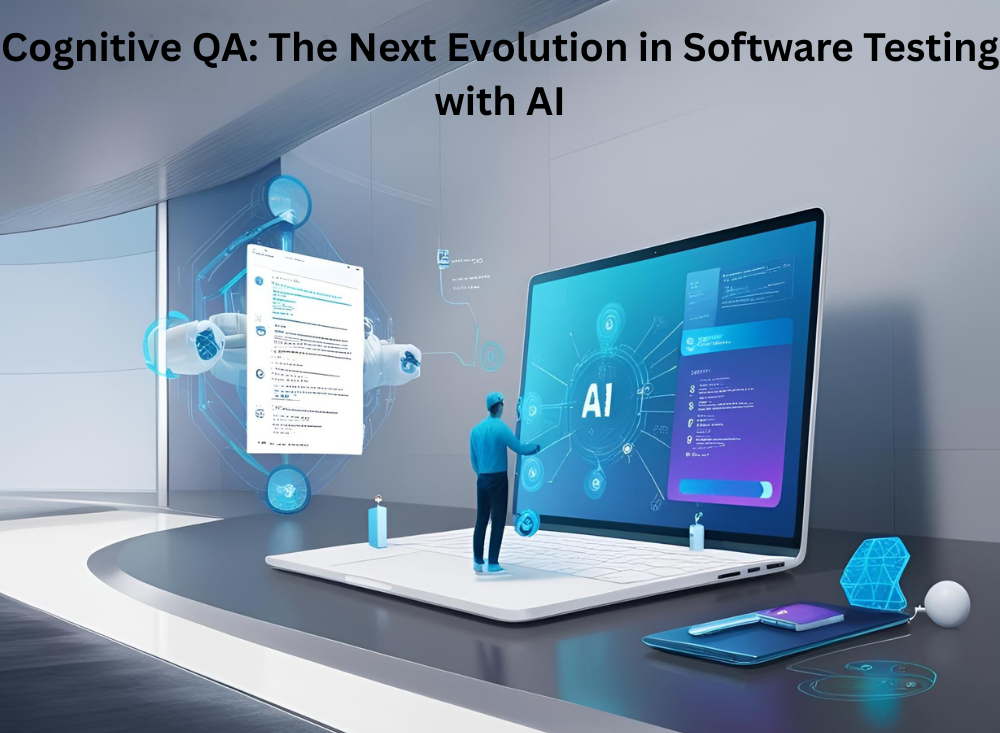
In the ever-accelerating digital landscape, conventional QA practices are struggling to keep pace with rapid releases, complex user experiences, and sprawling ecosystems of applications and APIs. As organizations push toward hyperautomation and smarter delivery pipelines, the concept of Cognitive QA has emerged as the next frontier in intelligent software testing.
Cognitive QA leverages the full spectrum of artificial intelligence — machine learning, natural language processing, predictive analytics, and reasoning — to go beyond traditional automation. It introduces a new paradigm where software can understand, learn, adapt, and recommend testing strategies much like a human would — but at machine scale and speed.
What is Cognitive QA?
Unlike conventional test automation that relies on scripted inputs and fixed outcomes, Cognitive QA mimics the way a human tester thinks. It understands the context of an application, analyzes test cases semantically, learns from past failures, and evolves continuously.
Core capabilities include:
- Contextual understanding of requirements and test documentation
- Self-healing test scripts that adapt to UI or logic changes
- AI-generated test cases based on user behavior analytics
- Continuous learning from production feedback and test outcomes
- Predictive insights into defect trends and potential regressions
In essence, Cognitive QA fuses the precision of machines with the intuition of human testers, creating smarter, more responsive QA processes.
Key Components of a Cognitive QA Framework
1.Natural Language Processing (NLP)
Using NLP, Cognitive QA tools can parse user stories, requirements, and even emails to auto-generate relevant test cases — bridging the gap between business and engineering.
2.Machine Learning Models
Historical test data is fed into ML models to spot defect trends, suggest areas of risk, and prioritize test execution accordingly. Over time, the system becomes better at predicting where bugs are most likely to appear.
3.Cognitive Automation
This refers to autonomous test design, execution, and result analysis. These systems don’t just run tests — they reason through them, adapting test flows dynamically in real time based on application behavior.
4.Visual and Behavioral Testing
Cognitive QA platforms often incorporate visual validation tools and user journey simulations to ensure that changes don’t impact the perceived quality of the app — something traditional automation may miss.
Why Cognitive QA Matters Now
🔹Faster Releases with Confidence
Modern software development is agile, fast, and iterative. Cognitive QA brings intelligent testing that scales with rapid changes — enabling faster releases without compromising quality.
🔹Reducing Manual Overhead
By learning and adapting continuously, these systems reduce the need for manual intervention in test creation and maintenance — freeing up QA teams to focus on exploratory and creative testing.
🔹Smart Test Prioritization
Instead of running thousands of regressions blindly, Cognitive QA can prioritize tests based on code changes, usage frequency, and defect probability — optimizing for both time and coverage.
Use Cases Across Industries
- Finance: Predicting where bugs may appear in high-risk workflows like transactions or reporting.
- Retail: Simulating diverse user behaviors during seasonal surges or A/B tests.
- Healthcare: Ensuring compliance and stability in applications handling sensitive patient data.
- Enterprise SaaS: Automatically adapting test cases to evolving UI/UX designs and new features.
Platforms Powering the Shift
Leading platforms such as Genqe.ai are pioneering the adoption of Cognitive QA with solutions that integrate seamlessly into DevOps pipelines. These platforms use AI to dynamically assess quality metrics, generate intelligent test scenarios, and provide real-time insights into system health — all while learning from each test cycle.
By harnessing Genqe.ai capabilities, organizations can transition from reactive to proactive quality assurance, identifying issues long before they affect users.
The Future of QA is Cognitive
As software becomes more intelligent, interconnected, and user-driven, testing must evolve to match its complexity. Cognitive QA represents a move toward human-in-the-loop systems, where AI assists testers in making faster, smarter, and more informed decisions.
Far from replacing QA professionals, Cognitive QA amplifies their abilities — automating the repetitive while elevating the creative and strategic.
Conclusion: Embracing the Intelligent QA Revolution
In 2025 and beyond, businesses that embrace Cognitive QA will lead the race in digital quality and resilience. With AI as a co-pilot, QA shifts from a bottleneck to a competitive advantage, accelerating releases, reducing costs, and delighting users.
Whether you’re modernizing legacy testing frameworks or launching a new product at scale, integrating platforms like Genqe.ai into your pipeline could be the smartest next step toward cognitive, context-aware quality assurance.