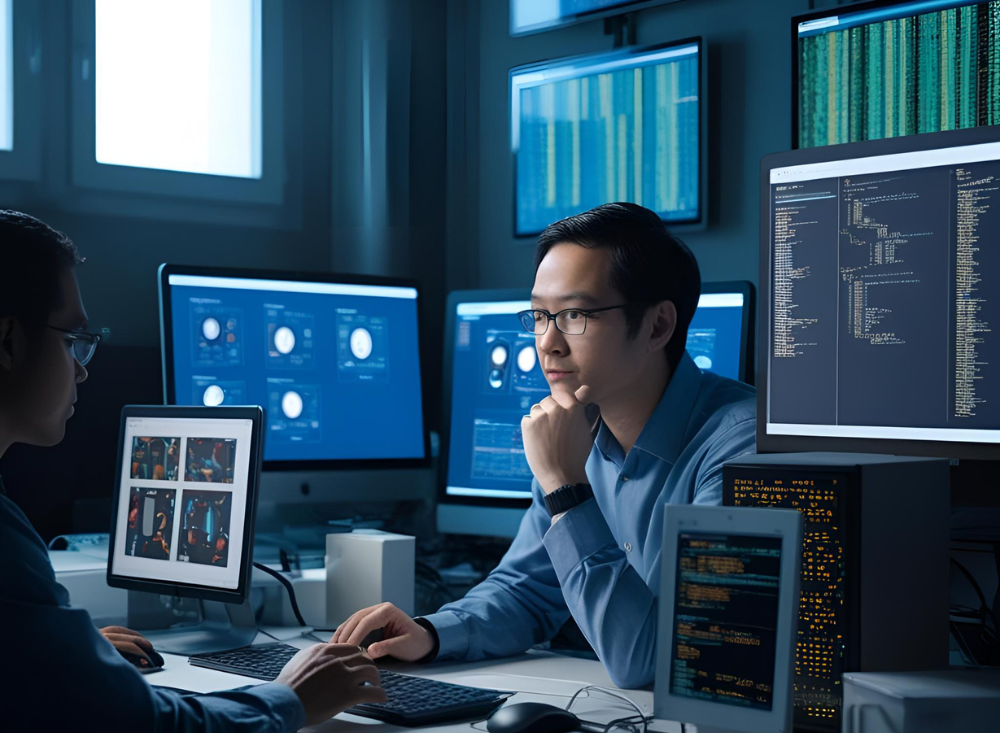
As artificial intelligence becomes deeply embedded in everything from healthcare diagnostics to loan approvals and recruitment tools, one issue continues to dominate the conversation: bias. AI systems are only as objective as the data they learn from, and when left unchecked, even the most sophisticated models can perpetuate or even amplify existing inequalities.
In 2025, testing for AI bias isn’t just a regulatory box to tick — it’s a moral and strategic imperative. Fairness, explainability, and accountability must be woven into every stage of the AI lifecycle. Fortunately, with the rise of intelligent QA platforms and testing tools, organizations now have ways to detect, measure, and mitigate bias before it causes harm.
Why Bias Happens in AI
AI bias typically stems from one or more of the following:
- Skewed training data: Historical data often contains embedded societal biases, especially in areas like criminal justice or hiring.
- Underrepresentation: If certain demographics are underrepresented in the training set, the model may fail to generalize to them effectively.
- Labeling inconsistencies: Human labelers may inject personal bias, even unintentionally.
- Feedback loops: Models deployed in the real world can perpetuate and reinforce bias if not monitored and retrained appropriately.
What makes bias particularly dangerous is its subtlety. It can go undetected for months or years until someone is denied a mortgage, misdiagnosed, or unfairly rejected from a job.
Key Areas to Focus When Testing for Bias
1.Data Auditing and Preprocessing
The first line of defense is a thorough audit of the training dataset. QA testers must look for:
- Imbalanced representation across gender, race, age, geography, etc.
- Correlated features that may indirectly encode sensitive attributes.
- Labeling biases or inconsistencies in subjective tasks (e.g., content moderation, facial recognition).
Automated tools using clustering and statistical analysis can help flag suspicious patterns early in the lifecycle.
2.Model Behavior Analysis
Once a model is trained, testers must evaluate its output across different segments. This includes
- Disparate impact analysis: Are error rates or predictions skewed for specific groups?
- Equal opportunity metrics: Do true positive rates differ across demographics?
- Counterfactual fairness: Would the outcome have changed if only a sensitive attribute (like race or gender) had been different?
Bias testing frameworks and fairness dashboards can visually highlight model disparities in real time.
3.Explainability and Transparency
Understanding why a model made a decision is key to detecting bias. Techniques like SHAP, LIME, and Integrated Gradients can surface the most influential features behind each prediction.
These insights allow QA teams to assess whether decisions are being driven by ethical, logical signals — or by proxies for sensitive characteristics.
4.Post-Deployment Monitoring
Bias isn’t a static issue. Once deployed, AI models interact with new data and user feedback, which can shift their behavior over time.
Continuous fairness testing is essential, using AI observability tools that flag performance degradation, ethical drift, or new disparities as they emerge in production.
How AI Itself Can Help Fight AI Bias
Ironically, AI can also help solve its own problems. With intelligent test generation, anomaly detection, and adaptive monitoring, modern AI testing platforms are capable of:
- Generating synthetic test cases to probe fairness across edge demographics.
- Clustering model behavior to detect outlier decisions.
- Suggesting debiasing strategies, such as reweighting data or adversarial training.
Genqe.ai, for instance, empowers QA teams to run fairness evaluations alongside functional and performance tests — automating the detection of bias across data pipelines and ML models without slowing down deployment.
Challenges Ahead
Despite advances in AI fairness testing, several challenges remain:
- No universal definition of fairness: Different use cases may require different fairness metrics, and cultural norms vary globally.
- Trade-offs with accuracy: Improving fairness can sometimes mean compromising raw accuracy or efficiency — decisions that must be carefully weighed.
- Data access: Ethical testing requires demographic and sensitive data, which often comes with privacy constraints and governance concerns.
The solution lies in collaborative AI development, where data scientists, QA engineers, ethicists, and legal experts work together to define acceptable boundaries.
Conclusion: Ethics as a QA Priority
Bias in AI is not a side effect — it’s a direct consequence of choices made during development and testing. As such, ethical QA is now a core responsibility, not just for AI teams but for businesses deploying these technologies.
In 2025, the most successful AI applications won’t be the fastest or the flashiest — they’ll be the fairest. They’ll win user trust, comply with global regulations, and reflect the values of the communities they serve.
Platforms like Genqe.ai, which combine intelligent automation with ethical insight, are helping organizations lead this charge — ensuring that AI not only works, but works fairly for everyone.