In the ever-evolving landscape of engineering, the acceleration of innovation is palpable, fueled by relentless technological progress and shifting market demands. Particularly within software engineering, a transformative shift is underway, steering practices towards greater agility, intelligence, and efficiency. This evolution is essential not only for enhancing product quality but also for meeting the complex requirements of contemporary systems and applications.
Traditional methods of software testing and quality assurance are increasingly proving inadequate in this dynamic new environment. As engineers strive to innovate more rapidly, reduce errors, and boost efficiency, the integration of Artificial Intelligence (AI) into software testing processes is emerging as a pivotal trend. This blog post delves into the significant challenges currently faced by the engineering sector, introduces the revolutionary role of AI in engineering, and explores how AI-powered tools like GenQE are becoming indispensable in the quest for superior software quality.
By the end of this article, you will gain a comprehensive understanding of the current engineering landscape, the impact of AI on software quality processes, and how leveraging these advanced technologies can lead to more robust, efficient, and innovative engineering practices.
The Challenges in Modern Engineering
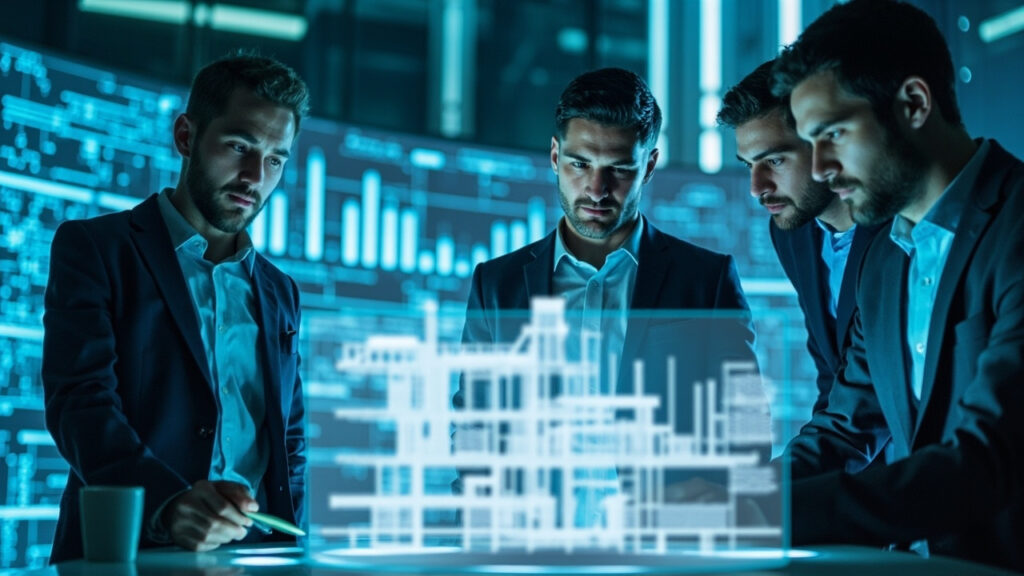
Engineering teams worldwide are grappling with several critical challenges that are compounded by the increasing complexity of projects, accelerated development cycles, heightened expectations for quality and reliability, and significant resource constraints. These challenges necessitate a reevaluation of traditional methodologies that are struggling to keep pace, thus impacting overall efficiency and quality.
Increasing Complexity of Projects
Today’s engineering projects are characterized by their vast scale and intricacy, which demand more than what conventional tools and methods can offer. This complexity not only complicates project management but also heightens the likelihood of errors that can be costly and time-consuming to rectify. The integration of AI, such as GenQE’s AI-driven test generation, can significantly mitigate these risks by enhancing the precision and coverage of tests, thus addressing the intricate demands of modern projects.
Accelerated Development Cycles
The modern market’s demand for speed has put immense pressure on engineering teams to expedite development cycles without compromising output quality. Traditional practices often fall short in this regard, creating a contradiction between the need for quick delivery and the imperative of high-quality results. Tools like GenQE aid in reconciling this contradiction by optimizing test execution and integrating seamlessly with continuous integration/continuous deployment (CI/CD) pipelines, thereby accelerating development without sacrificing quality.
High Expectations for Quality and Reliability
As technology becomes more embedded in daily life, expectations for system reliability and performance skyrocket. Engineering defects can lead to severe safety risks, substantial financial losses, and damage to brand reputation, making high-quality outputs essential. The predictive maintenance capabilities of AI and the comprehensive test coverage provided by platforms like GenQE play a crucial role in meeting these high expectations.
Resource Constraints
Despite the escalating demands, many engineering teams operate under significant resource constraints, with gaps between workload and available manpower or tools. AI’s ability to automate repetitive tasks and predict issues before they arise presents a solution to this dilemma, enabling more efficient resource management and reducing the burden on engineering teams.
The Role of AI in Modern Engineering
The integration of AI into engineering processes is revolutionizing how professionals tackle these challenges. AI offers a suite of tools and methodologies that automate routine tasks, predict potential issues, and deliver insights that are beyond human capabilities.
Enhanced Decision-Making
AI algorithms excel in analyzing vast amounts of data quickly and accurately, aiding in more informed decision-making. This capability is invaluable for identifying potential flaws or efficiencies early in the development process, thereby enabling corrective measures that save time and resources.
Automation of Repetitive Tasks
AI’s prowess in automating repetitive, time-consuming tasks allows engineering teams to devote more attention to complex and innovative aspects of product development. This not only expedites the development process but also minimizes the likelihood of human error, thus enhancing the overall quality of the engineering output.
Predictive Maintenance
In software engineering, AI’s predictive capabilities are particularly impactful. By foreseeing system failures or bugs before they manifest, AI-driven tools like GenQE ensure higher system uptime and enhance user satisfaction. This proactive approach to maintenance is crucial for maintaining the reliability and performance that users expect.
Introducing GenQE: AI-Powered Software Quality Engineering
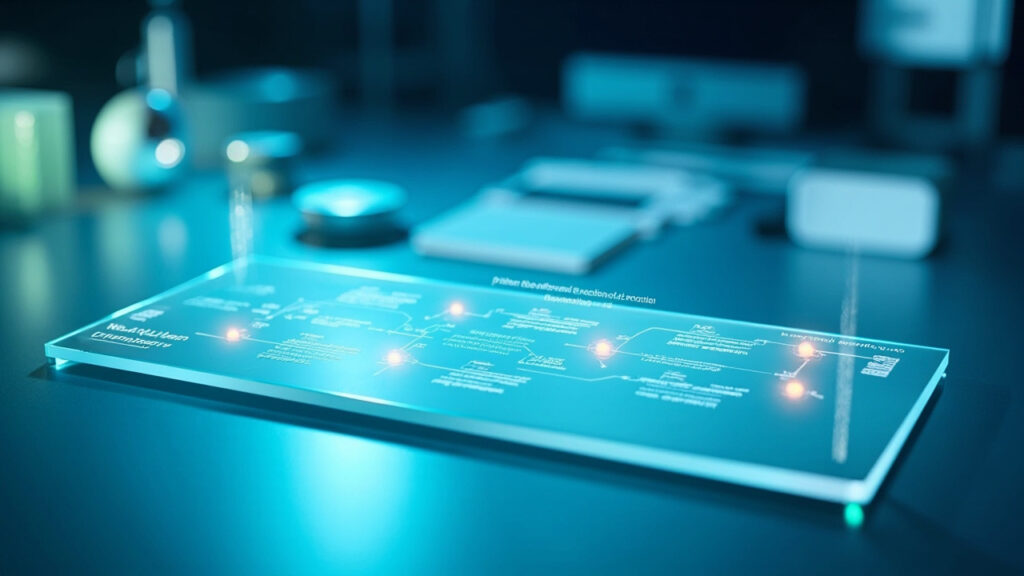
In discussing AI’s role in engineering, it is essential to highlight specific tools that exemplify this integration. GenQE stands out as a pioneering AI-powered software testing platform that effectively addresses the challenges previously outlined.
AI-Driven Test Generation
GenQE’s automation of test case creation significantly reduces the manual effort involved, thereby enhancing both the coverage and efficiency of tests. This feature proves particularly beneficial in managing the increased complexity of modern software applications, ensuring that even the most intricate functionalities are thoroughly tested.
Smart Test Execution
GenQE’s strategic approach to test execution, which involves prioritizing test cases based on risk analysis, ensures that critical areas receive attention first. This optimization of testing efforts focuses resources where they are most needed, making the testing process not only faster but also more effective.
Self-Healing Automation
The platform’s self-healing capabilities are a key feature, automatically adjusting test scripts when UI elements change. This functionality significantly reduces downtime and enhances the reliability of CI/CD pipelines, ensuring that software updates and deployments can occur smoothly and without interruption.
Comprehensive Test Coverage with GenQE
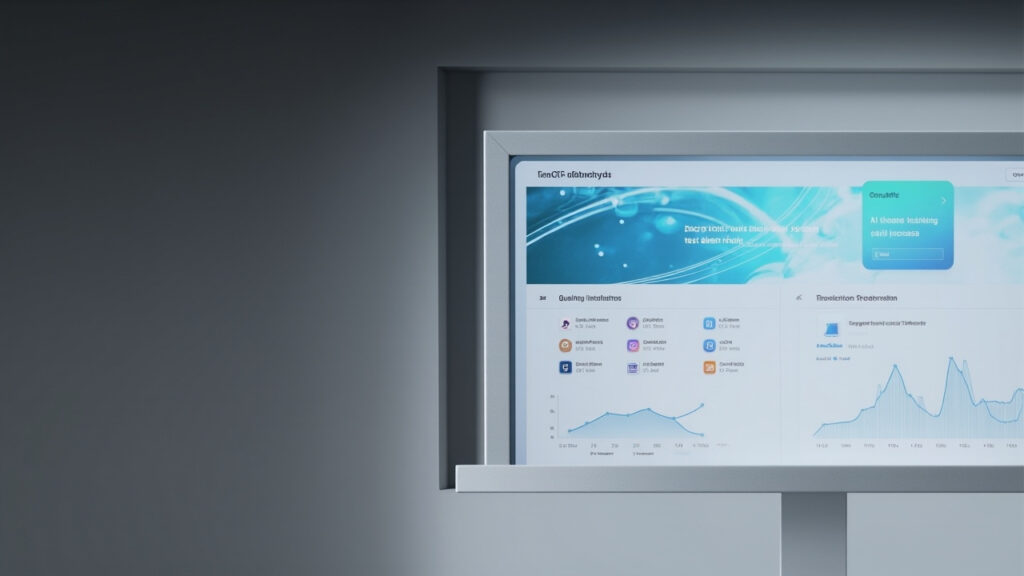
Achieving comprehensive test coverage is essential for ensuring that applications function seamlessly across different devices and platforms. GenQE provides extensive coverage that supports web, mobile, API, and cloud environments, making it an indispensable tool for modern engineering teams.
Multi-Platform Support
GenQE’s ability to test across various platforms ensures that applications deliver a consistent user experience, regardless of the device or operating system. This is particularly important in today’s diverse tech landscape, where users may access applications through a myriad of devices.
AI-Powered Defect Detection
Utilizing machine learning, GenQE identifies patterns in test results to detect anomalies and potential defects early. This early detection is key to preventing critical issues from reaching production, thus safeguarding the quality and reliability of the software.
Seamless Integration with DevOps & CI/CD
GenQE’s smooth integration with existing DevOps and CI/CD tools like Jenkins, GitHub Actions, and Azure DevOps enables automated testing to become a seamless part of the development pipeline. This integration is crucial for maintaining a robust workflow and ensuring continuous delivery of high-quality software.
The Impact of AI on Cost, Efficiency, and Quality in Engineering
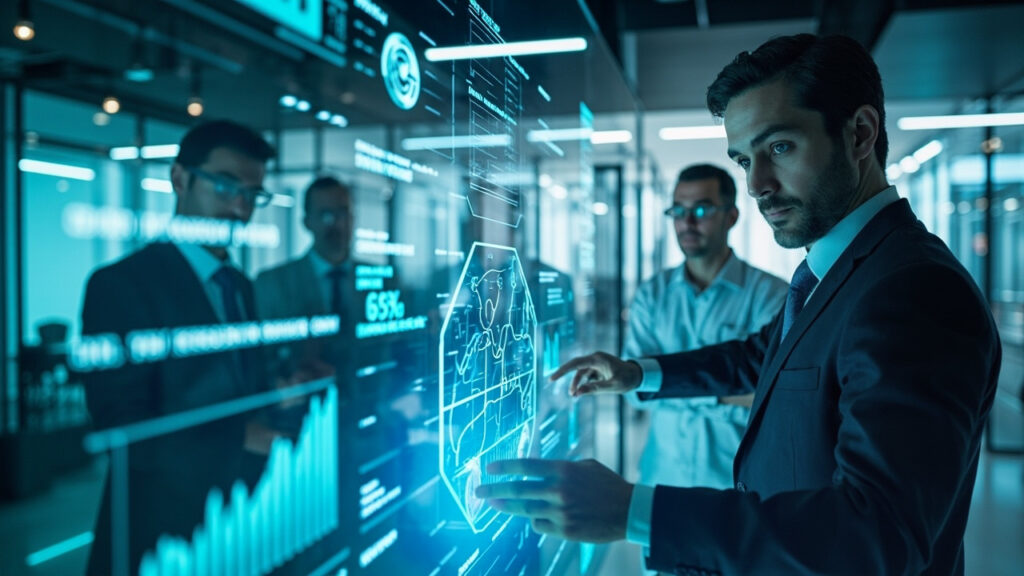
The adoption of AI-driven tools like GenQE brings tangible benefits in terms of cost savings, improved efficiency, and enhanced product quality.
Cost-Effectiveness
Automating repetitive testing tasks not only speeds up the release cycles but also significantly reduces the labor costs associated with manual testing. Early defect detection further prevents expensive fixes and recalls, contributing to overall cost reductions.
Enhanced Accuracy and Reliability
The precision of AI-driven testing minimizes the risks of human error, leading to more accurate and reliable software products. This reliability is critical for maintaining user trust and satisfaction.
Scalability
AI tools easily adapt to project scales, whether small startups or large enterprises. This scalability ensures that as project requirements grow, the testing processes can grow alongside them without a loss in quality or efficiency.
Conclusion: Embracing AI in Engineering for Future Success
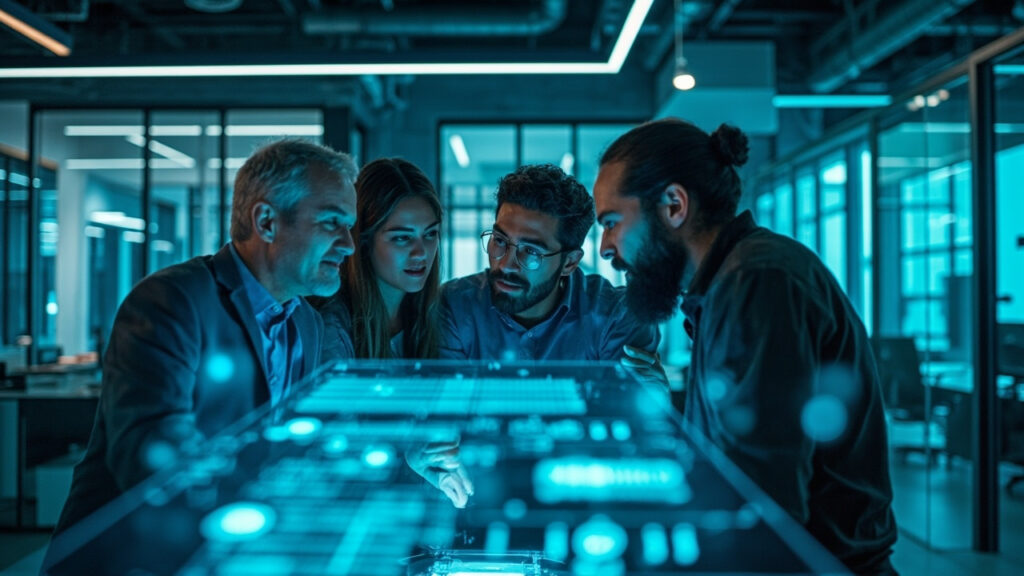
As we have explored, the integration of AI into engineering, particularly through tools like GenQE, offers a powerful solution to many of the challenges faced by today’s engineers. These technologies not only enhance the efficiency and accuracy of engineering processes but also enable teams to meet the high-quality standards demanded by modern users.
For engineering teams looking to stay competitive and innovative, adopting AI-powered tools is no longer just an option—it’s a necessity. As we move forward, the capabilities of AI in engineering will continue to expand, bringing even more sophisticated tools and methodologies to the forefront of this dynamic field.
If you’re aiming to enhance your engineering processes, exploring AI solutions like GenQE might just be your next best step. Embrace the future of engineering, and let AI take your projects to new heights of quality and efficiency.
Discover More Innovative Solutions
Want to learn more about the tools and technologies discussed in this article? Explore how these innovations can be tailored to your specific needs and workflow requirements.
Our team of experts is available to answer your questions and provide personalized insights into how modern solutions like GenQE can address your specific challenges.