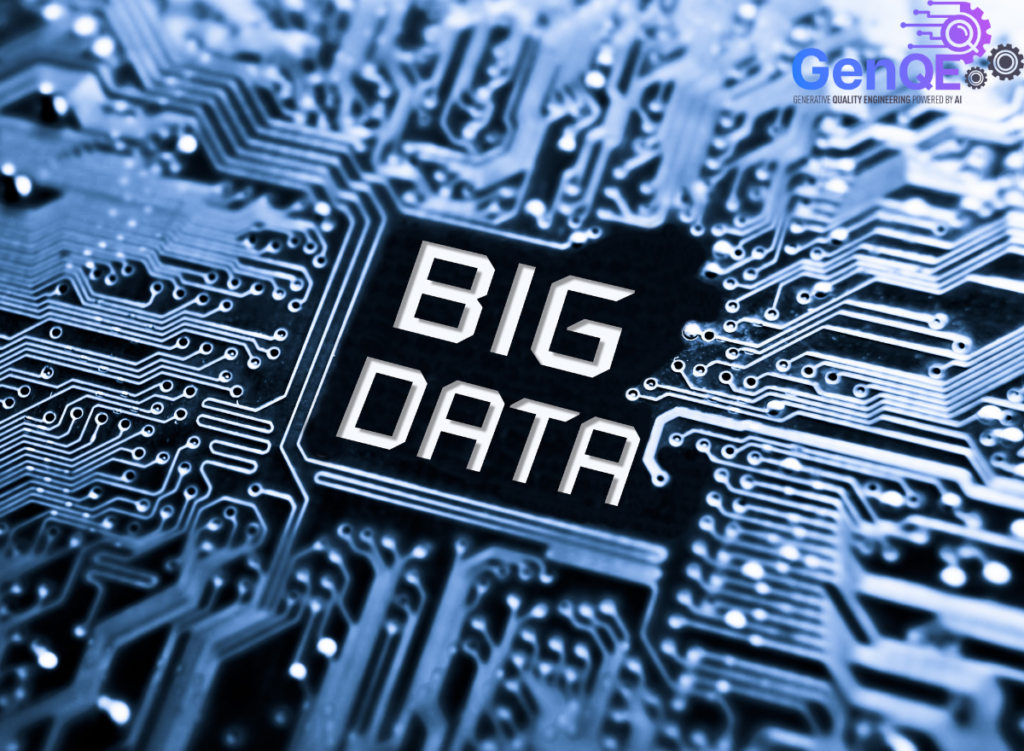
Big Data has become a cornerstone of modern business operations, enabling organizations to analyze vast amounts of data to drive decision-making, improve customer experiences, and optimize processes. However, ensuring the accuracy, reliability, and performance of Big Data systems is a complex challenge. Genqe.ai, a cutting-edge test orchestration platform, provides specialized tools and features to streamline Big Data testing and ensure the integrity of data pipelines, analytics, and storage systems.
What is Big Data Testing?
Big Data testing involves validating the functionality, performance, and reliability of systems that process and analyze large volumes of structured and unstructured data. It ensures that data is ingested, processed, stored, and analyzed correctly, and that the system can handle high data volumes, velocities, and varieties.
Challenges in Big Data Testing
- Data Volume: Testing systems that handle terabytes or petabytes of data requires specialized tools and infrastructure.
- Data Variety: Big Data systems process diverse data types, including structured, semi-structured, and unstructured data.
- Data Velocity: Real-time data processing systems must be tested for speed and accuracy.
- Complex Pipelines: Big Data pipelines involve multiple stages, such as data ingestion, transformation, and storage, each requiring thorough validation.
- Performance and Scalability: Ensuring that the system can handle high data loads and scale efficiently is critical.
How Genqe.ai Simplifies Big Data Testing
Genqe.ai is a powerful test orchestration platform designed to address the unique challenges of Big Data testing. Here’s how it can help:
1. Data Validation Testing
Ensuring the accuracy and completeness of data is a core aspect of Big Data testing. Genqe.ai enables:
- Data Integrity Checks: Validates that data is not corrupted or lost during ingestion, transformation, or storage.
- Schema Validation: Ensures that data conforms to predefined schemas and formats.
- Data Comparison: Compares source and target data to verify consistency and accuracy.
2. Performance and Scalability Testing
Big Data systems must handle high data volumes and velocities. Genqe.ai supports:
- Load Testing: Simulates high data loads to evaluate system performance and identify bottlenecks.
- Stress Testing: Pushes the system to its limits to assess its stability and scalability.
- Latency Testing: Measures the time taken for data processing and ensures it meets performance requirements.
3. Pipeline Testing
Big Data pipelines involve multiple stages, each requiring validation. Genqe.ai provides:
- End-to-End Testing: Validates the entire data pipeline, from ingestion to storage and analysis.
- Transformation Testing: Ensures that data transformations (e.g., aggregation, filtering) are performed correctly.
- Error Handling: Tests how the pipeline handles errors, such as missing or invalid data.
4. Real-Time Data Testing
Real-time data processing systems require specialized testing. Genqe.ai enables:
- Streaming Data Validation: Tests the accuracy and timeliness of real-time data streams.
- Event Processing Testing: Validates that events are processed and analyzed correctly in real-time.
5. Data Security and Compliance Testing
Protecting sensitive data is critical in Big Data systems. Genqe.ai provides:
- Encryption Testing: Ensures that data is encrypted during transmission and storage.
- Access Control Testing: Validates that only authorized users can access sensitive data.
- Compliance Testing: Ensures adherence to data protection regulations like GDPR and HIPAA.
6. AI-Driven Test Optimization
Genqe.ai leverages artificial intelligence to optimize Big Data testing:
- Smart Test Case Prioritization: Identifies and prioritizes high-impact test cases to ensure critical functionalities are tested first.
- Predictive Analytics: Detects potential issues before they impact the system, reducing downtime and improving reliability.
7. Integration with Big Data Tools
Genqe.ai integrates seamlessly with popular Big Data tools and frameworks, such as Hadoop, Spark, and Kafka, enabling:
- Automated Testing: Automates test execution across different stages of the Big Data pipeline.
- Tool-Specific Validation: Provides specialized tests for tools like HDFS, Hive, and HBase.
8. Real-Time Monitoring and Reporting
Genqe.ai offers real-time insights into test execution:
- Live Dashboards: Provides a visual overview of test progress, failures, and performance metrics.
- Detailed Reports: Generates comprehensive reports for stakeholders, enabling data-driven decision-making.
Benefits of Using Genqe.ai for Big Data Testing
- Improved Data Accuracy: Ensures that data is processed and stored correctly, reducing the risk of errors.
- Enhanced Performance: Validates that the system can handle high data volumes and velocities efficiently.
- Faster Time-to-Insights: Automates testing processes, enabling faster delivery of actionable insights.
- Cost Efficiency: Reduces manual effort and optimizes resource utilization.
- Regulatory Compliance: Ensures adherence to data protection regulations and provides audit-ready documentation.
Conclusion
Big Data testing is essential to ensure the accuracy, reliability, and performance of systems that process and analyze large volumes of data. Genqe.ai provides a comprehensive platform to streamline Big Data testing, offering specialized tools for data validation, performance testing, pipeline testing, and more. By leveraging Genqe.ai, organizations can confidently deploy Big Data systems that deliver accurate insights, meet performance requirements, and comply with regulatory standards. With Genqe.ai, Big Data testing becomes smarter, faster, and more efficient.